A Construction Method of Combined Air Quality Forecasting Model
An air quality and forecasting model technology, applied in neural learning methods, biological neural network models, forecasting, etc., can solve the problems of high forecast error, insensitive extreme value performance, and large forecast error in high-concentration pollution periods, and achieves the goal of reporting The effect of improving the rate, improving the forecasting effect, and improving the accuracy
- Summary
- Abstract
- Description
- Claims
- Application Information
AI Technical Summary
Problems solved by technology
Method used
Image
Examples
Embodiment 2011
[0078] Example From May 2011 to April 2012 PM in a certain place 10 Daily Mean Concentration Forecast
[0079] Prepare training sample data: PM of a local environmental automatic monitoring station from January 1, 2008 to April 30, 2012 10 Concentration data and weather monitoring and weather forecast data.
[0080] (1) Based on the training sample set, a BP neural network prediction model is established;
[0081] (11) Input layer neuron determination
[0082] Select the factors with larger comprehensive influence weights as the neurons in the input layer of the respective neural networks. Determine the input parameters of the PM10 daily average forecast model as: today's wind W p , Today's rainfall RF p , yesterday's rainfall RF p-1 , yesterday's air pressure AP p-1 , yesterday's relative humidity RH p-1 , Atmospheric stability level AS at 18:00 yesterday p-1 , yesterday's background concentration, and the previous day's background concentration.
[0083] (12) The n...
PUM
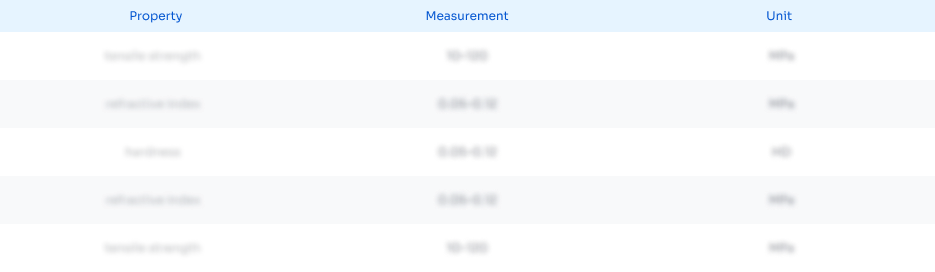
Abstract
Description
Claims
Application Information
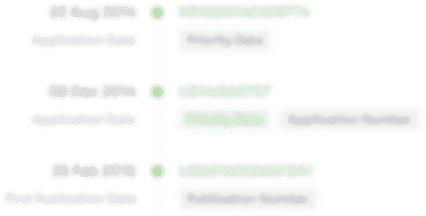
- R&D
- Intellectual Property
- Life Sciences
- Materials
- Tech Scout
- Unparalleled Data Quality
- Higher Quality Content
- 60% Fewer Hallucinations
Browse by: Latest US Patents, China's latest patents, Technical Efficacy Thesaurus, Application Domain, Technology Topic, Popular Technical Reports.
© 2025 PatSnap. All rights reserved.Legal|Privacy policy|Modern Slavery Act Transparency Statement|Sitemap|About US| Contact US: help@patsnap.com