A method for fabric defect detection
A defect detection and fabric technology, applied in the direction of optical testing defects/defects, etc., can solve the problems of affecting the degree of automation of the algorithm, noise interference, insufficient utilization of feature space, etc.
- Summary
- Abstract
- Description
- Claims
- Application Information
AI Technical Summary
Problems solved by technology
Method used
Image
Examples
specific Embodiment approach
[0035] The method of the invention mainly includes three steps of obtaining nonlinear mapping functions, learning optimal parameters and detecting defects. The specific implementation is as follows:
[0036] 1. The nonlinear mapping function calculation stage
[0037] For fabric images, the gray levels are often concentrated in a certain interval, rather than uniformly distributed in the entire gray space. If the general GLCM feature extraction method is used, linear quantization will be performed, and a large number of quantization values are not or rarely used, resulting in The generated GLCM matrix is very sparse and cannot fully reflect the texture features of the image. The present invention proposes nonlinear GLCM feature extraction. The basic idea is to quantify according to the occurrence probability of gray levels, refine the high-probability gray-scale intervals, and coarsely quantize the low-probability gray-scale intervals, so that image features can be effect...
PUM
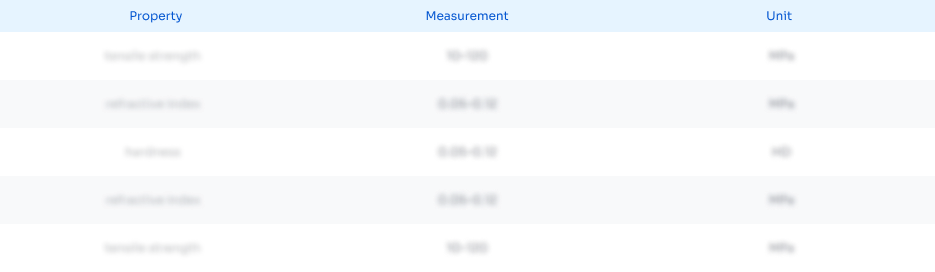
Abstract
Description
Claims
Application Information
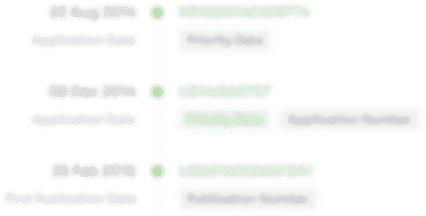
- R&D Engineer
- R&D Manager
- IP Professional
- Industry Leading Data Capabilities
- Powerful AI technology
- Patent DNA Extraction
Browse by: Latest US Patents, China's latest patents, Technical Efficacy Thesaurus, Application Domain, Technology Topic, Popular Technical Reports.
© 2024 PatSnap. All rights reserved.Legal|Privacy policy|Modern Slavery Act Transparency Statement|Sitemap|About US| Contact US: help@patsnap.com