High-dimensional data clustering method based on unweighted hypergraph segmentation
A technology of high-dimensional data and clustering methods, which is applied in the direction of instruments, character and pattern recognition, computer components, etc., can solve the problem that hypergraphs cannot reflect the data distribution of data sets, and achieve the effect of improving computing efficiency
- Summary
- Abstract
- Description
- Claims
- Application Information
AI Technical Summary
Problems solved by technology
Method used
Image
Examples
Embodiment
[0026] Such as figure 1 As shown, the clustering algorithm consists of three steps, which are the construction of unweighted hypergraph, the segmentation of unweighted hypergraph, and the evaluation of clustering results. The following are detailed introductions respectively.
[0027] 1. Construction of Unweighted Hypergraph S01
[0028] Define 1 node V
[0029] Map distinct attribute values in high-dimensional datasets to nodes of an unweighted hypergraph.
[0030] Definition 2 Hyperedge E
[0031] Each data record is mapped to a hyperedge of the unweighted hypergraph, and each node contained in the hyperedge is an attribute value of the data record.
[0032] The central idea of the unweighted hypergraph construction algorithm for high-dimensional datasets is to map different attribute values in high-dimensional datasets to nodes in unweighted hypergraphs, map each data record in high-dimensional datasets to a hyperedge, and The unweighted hypergraph...
PUM
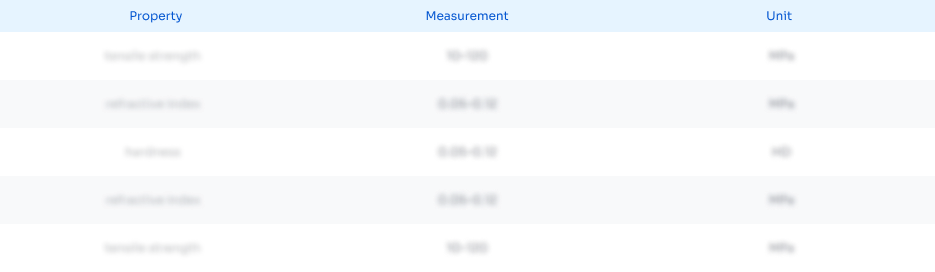
Abstract
Description
Claims
Application Information
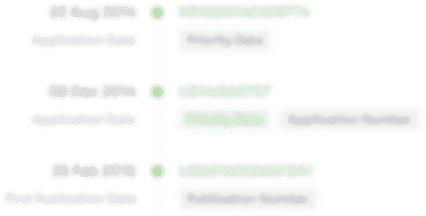
- R&D Engineer
- R&D Manager
- IP Professional
- Industry Leading Data Capabilities
- Powerful AI technology
- Patent DNA Extraction
Browse by: Latest US Patents, China's latest patents, Technical Efficacy Thesaurus, Application Domain, Technology Topic, Popular Technical Reports.
© 2024 PatSnap. All rights reserved.Legal|Privacy policy|Modern Slavery Act Transparency Statement|Sitemap|About US| Contact US: help@patsnap.com