Image super-resolution method based on active sampling and Gaussian process regression
A Gaussian process regression, active sampling technology, applied in the field of image super-resolution, can solve the problem of affecting the recognition of super-resolution reconstructed image details and reducing the quality of reconstruction.
- Summary
- Abstract
- Description
- Claims
- Application Information
AI Technical Summary
Problems solved by technology
Method used
Image
Examples
Embodiment Construction
[0045] The embodiments and effects of the present invention will be described in detail below in conjunction with the accompanying drawings.
[0046] refer to figure 1 , the implementation steps of the present invention are as follows:
[0047] Step 1, construct the initial training sample set T.
[0048] In the existing super-resolution methods, the training sample set comes from the super-resolution input image itself or external training images. The present invention uses external training images to construct a training sample set, and the specific process is:
[0049] (1a) Construct interpolation image I q with high-resolution image H q Composed of external training image pairs {I q ,H q}, where I q Interpolate images for bicubic Bicubic, H q is the corresponding high-resolution HR image, q=1,2,...,m, m is the number of training image pairs;
[0050] (1b) Randomly select n p×p training image patch pairs from m training image pairs
[0051] (1c) Extract the cent...
PUM
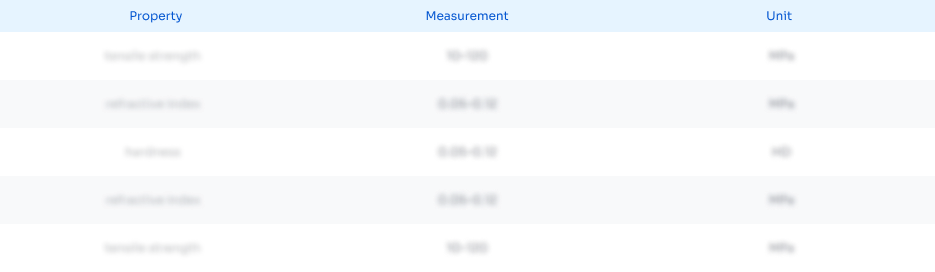
Abstract
Description
Claims
Application Information
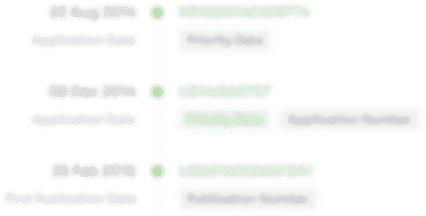
- R&D Engineer
- R&D Manager
- IP Professional
- Industry Leading Data Capabilities
- Powerful AI technology
- Patent DNA Extraction
Browse by: Latest US Patents, China's latest patents, Technical Efficacy Thesaurus, Application Domain, Technology Topic, Popular Technical Reports.
© 2024 PatSnap. All rights reserved.Legal|Privacy policy|Modern Slavery Act Transparency Statement|Sitemap|About US| Contact US: help@patsnap.com