Resource management method and system for large-scale distributed deep learning
A resource management and deep learning technology, applied in neural learning methods, resource allocation, electrical and digital data processing, etc., can solve problems such as low utilization of training system resources, inability to coordinate management of multiple resources, and out-of-bounds memory, and achieve reasonable guarantees. Configure, solve the missing gradient data, reduce the effect of memory consumption
- Summary
- Abstract
- Description
- Claims
- Application Information
AI Technical Summary
Problems solved by technology
Method used
Image
Examples
Embodiment Construction
[0058] In order to make the object, technical solution and advantages of the present invention clearer, the present invention will be further described in detail below in conjunction with the accompanying drawings and embodiments. It should be understood that the specific embodiments described here are only used to explain the present invention, not to limit the present invention.
[0059] First of all, explain and illustrate the technical terms involved in the present invention:
[0060] Parameter weight (weight): Since neurons are distributed layer by layer in the neural network structure, neurons in adjacent layers are connected to each other, and each connection has a parameter weight to determine the degree of influence of input data on neurons. The parameter weights are arranged in layers to form a parameter matrix, so that the forward calculation and backward calculation of each layer of the neural network can be expressed in the form of matrix multiplication.
[0061]...
PUM
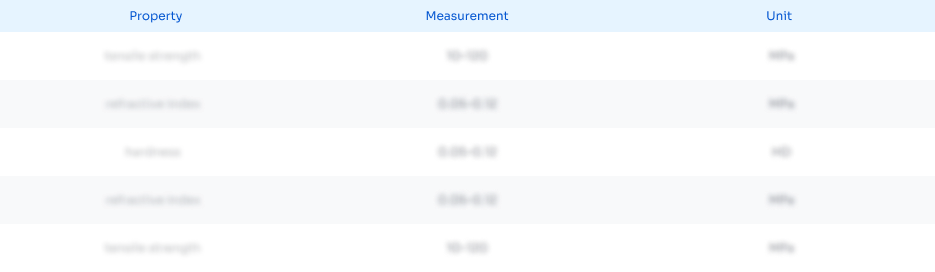
Abstract
Description
Claims
Application Information
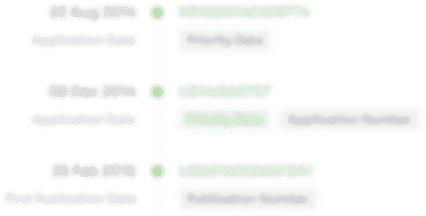
- R&D
- Intellectual Property
- Life Sciences
- Materials
- Tech Scout
- Unparalleled Data Quality
- Higher Quality Content
- 60% Fewer Hallucinations
Browse by: Latest US Patents, China's latest patents, Technical Efficacy Thesaurus, Application Domain, Technology Topic, Popular Technical Reports.
© 2025 PatSnap. All rights reserved.Legal|Privacy policy|Modern Slavery Act Transparency Statement|Sitemap|About US| Contact US: help@patsnap.com