LIBS multi-component quantitative inversion method based on deep learning convolutional neural network
A convolutional neural network and deep learning technology, applied in neural learning methods, biological neural network models, neural architectures, etc., can solve problems such as the inability to meet application requirements, the single core structure of CNN network, and the inability to guarantee accuracy.
- Summary
- Abstract
- Description
- Claims
- Application Information
AI Technical Summary
Problems solved by technology
Method used
Image
Examples
Embodiment Construction
[0057] In conjunction with a specific experimental case, the application process of the method described in the summary of the invention is illustrated below:
[0058] 1. In this experiment, there are 11 experimental samples in total, ie N=11, all of which are national standard substances, marked as No. 1 to No. 11 respectively. Standard samples No. 1 to No. 11 are: 1) clay 2) soft clay 3) carbonate rock 4) kaolin 5) basalt 6) pegmatite 7) dolomite 8) andesite 9) granite gneiss 10) Silica sandstone 11) Shale. In these 11 samples, there are 22 kinds of chemical components of main substances, that is, L=22. These components are numbered 0-21, and a general list of chemical components of substances is made. The chemical components corresponding to each component number are shown in Table 1. Show.
[0059] serial number Element serial number Element 0 SiO 2
11 Cl 1 Al 2 o 3
12 CO 2
2 Fe 2 o 3
13 h 2 o +
3 ...
PUM
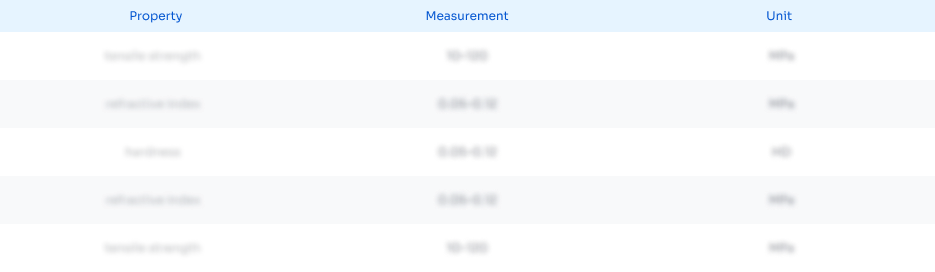
Abstract
Description
Claims
Application Information
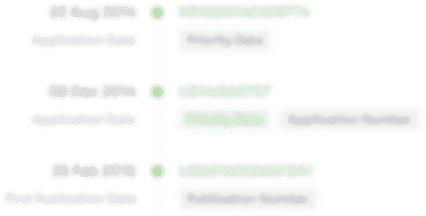
- R&D
- Intellectual Property
- Life Sciences
- Materials
- Tech Scout
- Unparalleled Data Quality
- Higher Quality Content
- 60% Fewer Hallucinations
Browse by: Latest US Patents, China's latest patents, Technical Efficacy Thesaurus, Application Domain, Technology Topic, Popular Technical Reports.
© 2025 PatSnap. All rights reserved.Legal|Privacy policy|Modern Slavery Act Transparency Statement|Sitemap|About US| Contact US: help@patsnap.com