High-resolution remote sensing image supervised segmentation method based on variable Gaussian hybrid model
A Gaussian mixture model and high-resolution technology, which is applied in the field of image processing, can solve the problems of inability to process high-resolution remote sensing image segmentation, does not consider the spatial relationship between pixels, and GMM cannot model accurately, so as to overcome the impact and improve the segmentation speed Fast, easy-to-achieve effects
- Summary
- Abstract
- Description
- Claims
- Application Information
AI Technical Summary
Problems solved by technology
Method used
Image
Examples
Embodiment Construction
[0045] The specific implementation manners of the present invention will be described in detail below in conjunction with the accompanying drawings.
[0046]A supervised segmentation method for high-resolution remote sensing images based on a variable Gaussian mixture model, such as figure 1 shown, including the following steps:
[0047] Step 1: Read the high-resolution remote sensing image to be segmented;
[0048] In this embodiment, the high-resolution remote sensing image domain to be segmented is defined X={x j ,j=1,...,n}, j is the pixel index, n is the total number of pixels, x j is the gray value of the jth pixel, the size of the high-resolution remote sensing image domain X to be segmented is 256×256, and the total number of pixels n=65536.
[0049] Step 2: Perform supervised sampling for each feature category in the high-resolution remote sensing image to be segmented, and calculate the frequency value of the gray value of each pixel in the corresponding feature c...
PUM
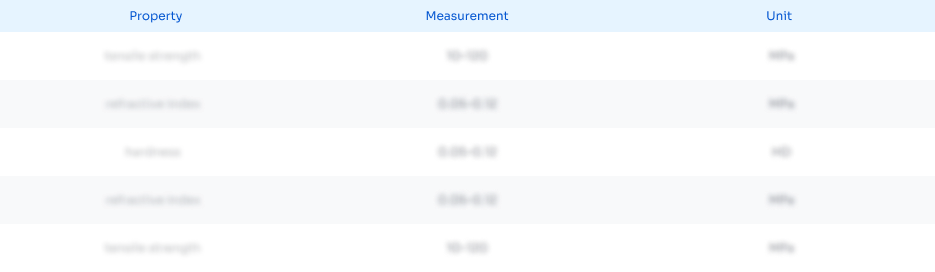
Abstract
Description
Claims
Application Information
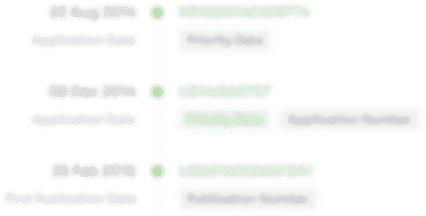
- R&D Engineer
- R&D Manager
- IP Professional
- Industry Leading Data Capabilities
- Powerful AI technology
- Patent DNA Extraction
Browse by: Latest US Patents, China's latest patents, Technical Efficacy Thesaurus, Application Domain, Technology Topic, Popular Technical Reports.
© 2024 PatSnap. All rights reserved.Legal|Privacy policy|Modern Slavery Act Transparency Statement|Sitemap|About US| Contact US: help@patsnap.com