Hyperspectral Image Classification Method Based on Neighbor Propagation Clustering and Sparse Multi-kernel Learning
A hyperspectral image and multi-core learning technology, which is applied in character and pattern recognition, instruments, calculations, etc., can solve the problems of difficult hyperspectral image data, high computational complexity of multi-core learning methods, and large kernel scale, to overcome time The effect of excessive complexity, avoiding the degradation of classification performance, and broad application prospects
- Summary
- Abstract
- Description
- Claims
- Application Information
AI Technical Summary
Problems solved by technology
Method used
Image
Examples
Embodiment Construction
[0030] refer to figure 1 , the implementation steps of the present invention are as follows:
[0031] Step 1, input a hyperspectral image, obtain training samples and test samples.
[0032] (1.1) Input hyperspectral image: The image contains l spectral bands and n samples;
[0033] (1.2) Randomly select 10% samples from these n samples to form a training sample set Use the remaining samples to form the test sample set Among them, pp and qq represent the number of training samples and test samples respectively, satisfying pp+qq=n;
[0034] Step 2, for the training sample set X pp and the test sample set X qq Perform column normalization operations respectively to obtain the training sample set X after column normalization p and the test sample set X q .
[0035] Step 3, use the normalized training sample set X p In the l bands, the Gaussian kernel matrix set K is constructed through m different kernel parameters.
[0036] (3.1) Extract training sample set X p The i...
PUM
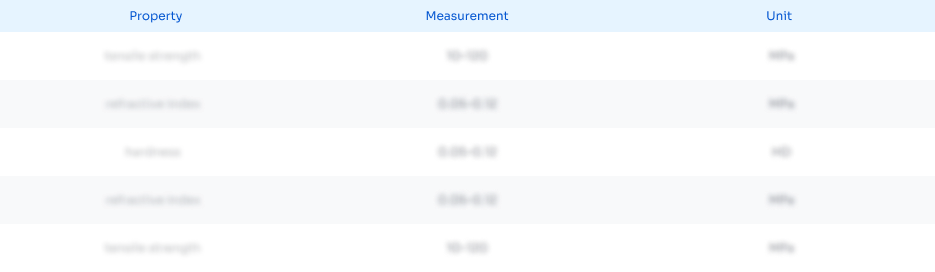
Abstract
Description
Claims
Application Information
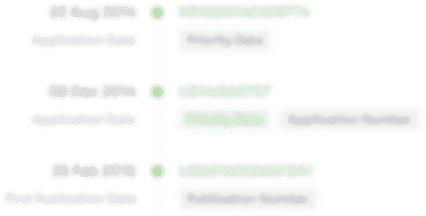
- R&D Engineer
- R&D Manager
- IP Professional
- Industry Leading Data Capabilities
- Powerful AI technology
- Patent DNA Extraction
Browse by: Latest US Patents, China's latest patents, Technical Efficacy Thesaurus, Application Domain, Technology Topic, Popular Technical Reports.
© 2024 PatSnap. All rights reserved.Legal|Privacy policy|Modern Slavery Act Transparency Statement|Sitemap|About US| Contact US: help@patsnap.com