Hyperspectral Image Classification Method Based on Spatial Information Migration
A hyperspectral image and spatial information technology, which is applied in the fields of instruments, character and pattern recognition, computer parts, etc., can solve the problems of slow classification speed, unsatisfactory classification effect, and a large number of labeled samples, so as to speed up the classification speed and improve the classification speed. Correct rate, the effect of reducing the number of bands
- Summary
- Abstract
- Description
- Claims
- Application Information
AI Technical Summary
Problems solved by technology
Method used
Image
Examples
Embodiment Construction
[0022] refer to figure 1 , the hyperspectral image classification method based on spatial information migration of the present invention, comprises the following steps:
[0023] Step 1: Input hyperspectral image I R×N , R is a band with a size of m×n in the spectrum, and N is the number of bands in the hyperspectral image. This hyperspectral image was taken by the ROSIS sensor at the University of Pavia, Italy, and the resolution of the image is 610×340. Grayscale images such as figure 2 As shown, there are a total of 9 types of ground objects in this picture, and the real distribution labels are as follows image 3 shown.
[0024] Step 2: Input hyperspectral image I R×N The labeled sample X in i and mark label Y i , X i is the feature vector of the i-th sample in the hyperspectral image, Y i is the same as the labeled sample X i corresponding marker label, Y i ∈ {1,2,...,k}, k is the number of categories in the hyperspectral image.
[0025] Step 3: Randomly select...
PUM
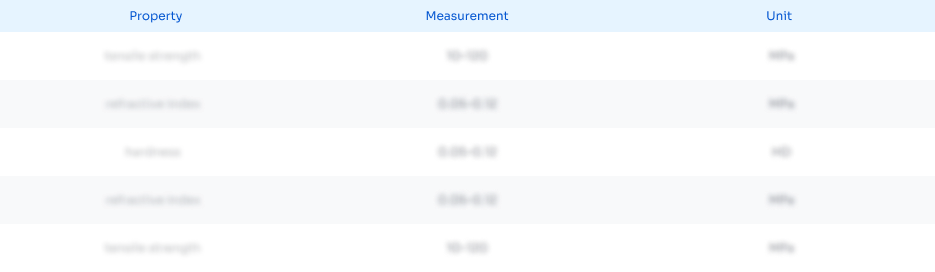
Abstract
Description
Claims
Application Information
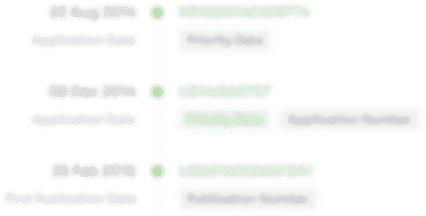
- R&D Engineer
- R&D Manager
- IP Professional
- Industry Leading Data Capabilities
- Powerful AI technology
- Patent DNA Extraction
Browse by: Latest US Patents, China's latest patents, Technical Efficacy Thesaurus, Application Domain, Technology Topic, Popular Technical Reports.
© 2024 PatSnap. All rights reserved.Legal|Privacy policy|Modern Slavery Act Transparency Statement|Sitemap|About US| Contact US: help@patsnap.com