An Incremental Trajectory Anomaly Detection Method Based on Incremental Kernel Principal Component Analysis
A nuclear principal component analysis and anomaly detection technology, which is applied in the directions of instruments, calculations, character and pattern recognition, etc., can solve the problems of high computational complexity, achieve the effect of improving efficiency and reducing computational complexity
- Summary
- Abstract
- Description
- Claims
- Application Information
AI Technical Summary
Problems solved by technology
Method used
Image
Examples
Embodiment 1
[0023] Embodiment 1: firstly carry out the initialization calculation of the model, use the traditional Batch KPCA to carry out the initial nuclear feature space calculation, whenever there are M new trajectory data coming, first standardize the M trajectory data; then use BatchKPCA to calculate the new The kernel feature space of augmented data. Calculate the average reconstruction error of the newly added data and the training data respectively. If the error of the two is greater than the given threshold, perform the subsequent nuclear feature space segmentation-merging method to update the nuclear feature space; then project the updated nuclear feature space , extract the principal components; finally use a class of support vector machines for unsupervised learning and anomaly detection.
[0024] see figure 1 As shown, an incremental trajectory anomaly detection method based on incremental kernel principal component analysis includes the following steps:
[0025] (1) Init...
Embodiment 2
[0037] Embodiment 2: the comparison of the present invention and the incremental trajectory abnormal detection algorithm based on traditional KPCA (Batch KPCA);
[0038] In order to verify the effectiveness of the present invention, a total of 221 trajectories from 7270 trajectory points in the Atlantic hurricane data between 1990 and 2006 were selected as the experimental data set for verification. From figure 2 It can be seen that the execution time of the traditional KPCA-based incremental trajectory anomaly detection method increases rapidly as the sliding data window increases. The execution time of the incremental trajectory anomaly detection algorithm based on kernel feature space segmentation-merging also increases with the increase of the sliding data window, but the magnitude is not large. And in the case of the same sliding data window size, the incremental trajectory anomaly detection algorithm based on kernel feature space segmentation-merging takes less computi...
Embodiment 3
[0039] Embodiment 3: An abnormality detection effect diagram of the present invention;
[0040] Select the detection results of the 4th iteration and the 6th iteration of the method of the present invention on the Atlantic hurricane data set, such as image 3 and Figure 4shown. The thicker lines in the figure represent detected abnormal trajectories, and the thinner lines represent normal trajectories. It can be seen from the figure that the anomaly detection effect is very good, and many trajectories with abnormal behavior have been detected. And from the results of two iterations, it can be seen that with the continuous addition of new trajectories, some new abnormal trajectories are detected, and because the kernel feature space is constantly updated, some earlier anomalous trajectories are ignored.
PUM
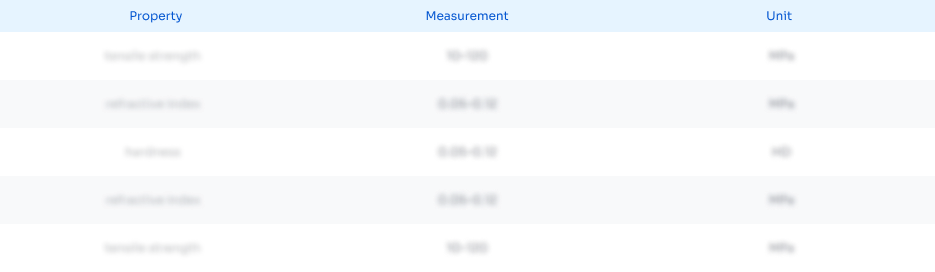
Abstract
Description
Claims
Application Information
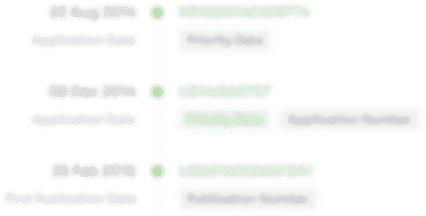
- R&D Engineer
- R&D Manager
- IP Professional
- Industry Leading Data Capabilities
- Powerful AI technology
- Patent DNA Extraction
Browse by: Latest US Patents, China's latest patents, Technical Efficacy Thesaurus, Application Domain, Technology Topic, Popular Technical Reports.
© 2024 PatSnap. All rights reserved.Legal|Privacy policy|Modern Slavery Act Transparency Statement|Sitemap|About US| Contact US: help@patsnap.com