Image classification method based on multi-task multi-instance support vector machine
A technology of support vector machine and classification method, which is applied in the field of image classification based on multi-task and multi-example support vector machine, which can solve the problems of affecting the effect of image classification, high artificial success, and performance degradation of classifiers.
- Summary
- Abstract
- Description
- Claims
- Application Information
AI Technical Summary
Problems solved by technology
Method used
Image
Examples
Embodiment Construction
[0016] The image classification method based on multi-task multi-example support vector machine of the present invention comprises the following steps:
[0017] The first step is to obtain several groups of images, and ensure that the number of images in each group is not large. Set up several learning tasks in units of groups, and perform manual classification of images in the form of manual marking. For example, if there are T groups of images, T image classifier learning tasks are established, and since the number of images for T tasks is not large, manual labeling can be performed.
[0018] In the second step, all images of all learning tasks are converted into multi-instance data. Since the image contains multiple scenes, only one of the key scenes is needed when classifying, so converting the entire image into a single example for classification may ignore the correlation of multiple scenes, resulting in a classification effect becomes worse, so at this time, a multi-in...
PUM
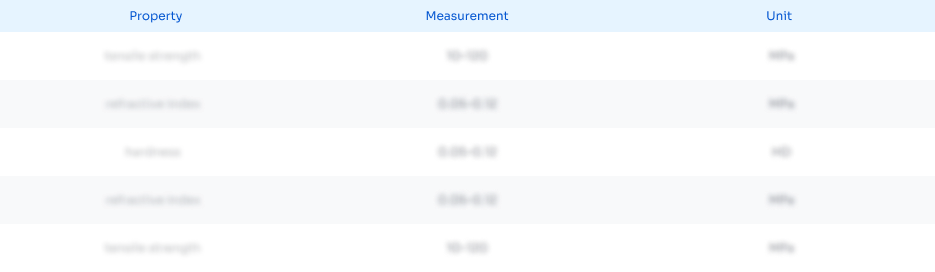
Abstract
Description
Claims
Application Information
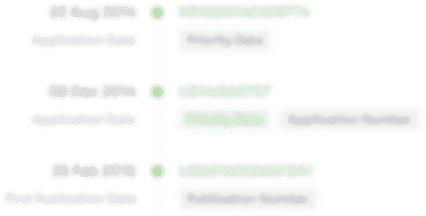
- R&D Engineer
- R&D Manager
- IP Professional
- Industry Leading Data Capabilities
- Powerful AI technology
- Patent DNA Extraction
Browse by: Latest US Patents, China's latest patents, Technical Efficacy Thesaurus, Application Domain, Technology Topic, Popular Technical Reports.
© 2024 PatSnap. All rights reserved.Legal|Privacy policy|Modern Slavery Act Transparency Statement|Sitemap|About US| Contact US: help@patsnap.com