Improved-support-vector-machine-based power load prediction correction method
A support vector machine and power load technology, applied in forecasting, instrumentation, data processing applications, etc., can solve problems such as inability to accurately reflect current and future changes in power demand, power demand development trends, and low accuracy of power load
- Summary
- Abstract
- Description
- Claims
- Application Information
AI Technical Summary
Problems solved by technology
Method used
Image
Examples
specific Embodiment approach 1
[0016] Specific implementation mode one: combine figure 1 The electric load forecast correction method based on the improved support vector machine of this embodiment is specifically prepared according to the following steps:
[0017] Step 1. Select sample data from the database or on-site;
[0018] Step 2: Encoding the sample data;
[0019] Step 3, preprocessing the encoded sample data;
[0020] Step 4, initialization kernel function; described initialization kernel function, because selection of different kernel functions can constitute different predictive models, so the selection of kernel function is also crucial; Select Gaussian radial basis kernel function to preprocessed Sample data for processing;
[0021] Step five, using the improved support vector machine to predict the electric load.
[0022] The effect of this implementation mode:
[0023] This embodiment relates to an electric load forecast correction method based on an improved support vector machine. The...
specific Embodiment approach 2
[0024] Embodiment 2: This embodiment differs from Embodiment 1 in that: the sample data is specifically the power grid operation competition data provided by the European Intelligent Technology Network. Other steps and parameters are the same as those in Embodiment 1.
specific Embodiment approach 3
[0025] Embodiment 3: The difference between this embodiment and Embodiment 1 or 2 is that the training samples for encoding the sample data are as follows: short-term power load forecasting is not just a matter of using a single factor to predict future loads, Its training samples are composed of multiple factors and are a multi-dimensional non-linear sample set; including historical load, temperature and holiday information. Other steps and parameters are the same as those in Embodiment 1 or Embodiment 2.
PUM
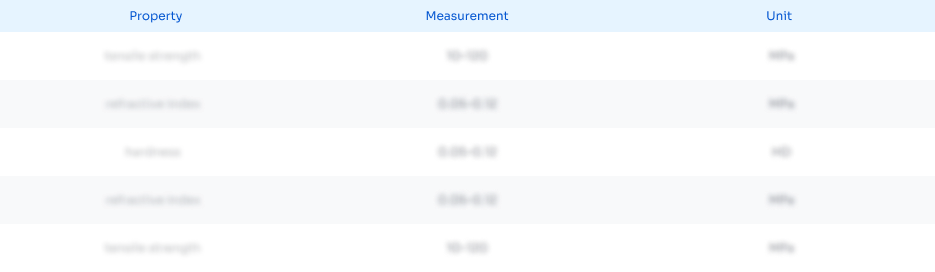
Abstract
Description
Claims
Application Information
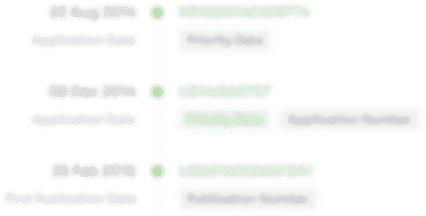
- R&D Engineer
- R&D Manager
- IP Professional
- Industry Leading Data Capabilities
- Powerful AI technology
- Patent DNA Extraction
Browse by: Latest US Patents, China's latest patents, Technical Efficacy Thesaurus, Application Domain, Technology Topic.
© 2024 PatSnap. All rights reserved.Legal|Privacy policy|Modern Slavery Act Transparency Statement|Sitemap