A method for modality synthesis of medical images
A medical image and modality technology, applied in image enhancement, image analysis, graphics and image conversion, etc., can solve the problems of limited universality, inability to guarantee the correspondence of individual images, approaching the mapping relationship of modality medical images, etc., and achieve prediction accuracy high effect
- Summary
- Abstract
- Description
- Claims
- Application Information
AI Technical Summary
Problems solved by technology
Method used
Image
Examples
Embodiment 1
[0054] A method for modality synthesis of medical images, comprising the following steps:
[0055] The first step is to normalize the spatial resolution of the source modal image. All the source modal images are spatially reduced by downsampling or enlarged by interpolation, so that the resolution of the image is normalized to same size;
[0056] In the second step, the gray value of the source modal image is standardized or normalized, and the gray value of the source modal image processed in the first step is normalized to a mean value of 0 and a standard deviation of 1;
[0057] In the third step, the processed source modal image is used as input, predicted by the convolutional neural network, and the two-dimensional data, three-dimensional volume data or adjacent layers in the source modal image data processed in the second step The image is input to predict the target modality image corresponding to the source modality, and the convolutional neural network architecture a...
Embodiment 2
[0097] see Figure 1-2 , a technical solution provided by this embodiment: the image data of a certain source modality is known, and the image synthesis of a corresponding target modality is predicted by the present invention, including the following basic steps:
[0098] (1) Normalize the spatial resolution of the source modal image, and reduce all the source modal images spatially by downsampling or enlarge them by interpolation, so that the resolution of the image is normalized to the same size, in this embodiment, the N3 algorithm is used to de-bias the source modality image (MR image); and then use the target modality image (CT image) of a certain patient as a template to convert all other source modality The image (MR image) and the image (CT image) of the target modality carry out image registration with the flirt algorithm; and the input image pixel size is normalized to 512 * 512, the present invention is based on the image produced by the existing imaging equipment, ...
Embodiment 3
[0142] A convolutional neural network method for medical image modality synthesis, other are the same as embodiment 1 or 2, specifically adopt the following features to carry out:
[0143] like Figure 4 As shown, the convolutional neural network prediction output is an image of the target modality (CT image) corresponding to the source modality image (MR image); firstly, the N3 algorithm is used to de-bias the source modality image (MR image) Processing; then using the target modality image (CT image) of a certain patient as a template, all other source modality images (MR images) and target modality images (CT images) are image-registered using the flirt algorithm; And normalize the input image pixel size to 512×512.
[0144] The convolutional network includes a convolutional layer with an image size of 512×512, and a convolutional layer with a size of 256×256, 128×128, 64×64, and 32×32 through pooling and downsampling in turn, except The convolution kernel size of the fir...
PUM
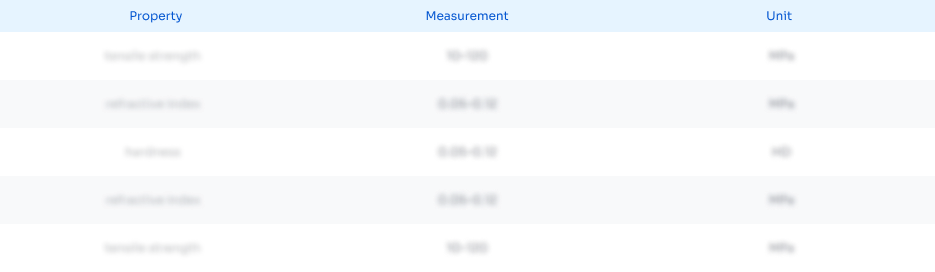
Abstract
Description
Claims
Application Information
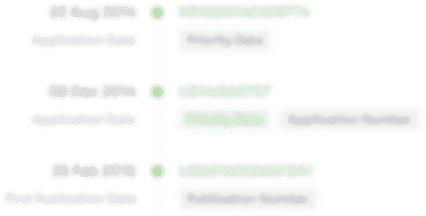
- R&D Engineer
- R&D Manager
- IP Professional
- Industry Leading Data Capabilities
- Powerful AI technology
- Patent DNA Extraction
Browse by: Latest US Patents, China's latest patents, Technical Efficacy Thesaurus, Application Domain, Technology Topic, Popular Technical Reports.
© 2024 PatSnap. All rights reserved.Legal|Privacy policy|Modern Slavery Act Transparency Statement|Sitemap|About US| Contact US: help@patsnap.com