ARIMA model and user regulation-based dynamic variance real-time alarming method
A dynamic variance and model technology, applied in special data processing applications, instruments, electrical and digital data processing, etc., can solve problems such as inability to achieve accurate early warning, threshold range fluctuations, and unreasonable fixed thresholds, and achieve the effect of improving the accuracy of early warning.
- Summary
- Abstract
- Description
- Claims
- Application Information
AI Technical Summary
Problems solved by technology
Method used
Image
Examples
Embodiment 1
[0026] In order to solve the above problems, the embodiment of the present invention uses the ARIMA (p, d, q) prediction model as the cornerstone, and designs a dynamic variance early warning mechanism applicable to various industries, see figure 1 and figure 2 , the method includes the following steps:
[0027] 101: Divide a period into different time periods with the same characteristics according to the data characteristics, and use the data characteristics of each period to determine the p, d, q and other parameters in the forecast model;
[0028] Among them, p is the order of autoregression, q is the order of moving average, and d is the order of difference.
[0029] 102: Establish different forecasting models for each time period;
[0030] This step can reduce the error caused by building a forecast model in all time periods.
[0031] 103: Design the whole early warning mechanism as two small prediction subsystems S1 and S2, and a comparison system C.
[0032] Among...
Embodiment 2
[0039] The scheme in embodiment 1 is further introduced below in conjunction with specific calculation formulas and examples, see the following description for details:
[0040] 201: Divide the data within a period into different periods with the same characteristics;
[0041] 202: Design the prediction subsystem S1, the steps are as follows:
[0042] 1) According to the data of different periods in the current cycle, establish an ARIMA(p,d,q) time prediction model that satisfies each period, including determining the appropriate p,d,q parameters;
[0043] 2) According to the ARIMA(p,d,q) time prediction model established in step 1), predict the forecast data of T sampling periods And record the real data X of the T sampling period at the same time;
[0044] 3) calculate
[0045] 203: Design the prediction subsystem S2, the steps are as follows:
[0046] 1) Calculate the variance of all real data in the same period corresponding to the first n periods of the current per...
Embodiment 3
[0054] The scheme in embodiment 1 and 2 is further introduced below in conjunction with specific calculation formula, example, see the following description for details:
[0055] Prediction subsystem 1: According to the data characteristics of each period, it may be assumed that the data collected in each period can be divided into four time periods A, B, C, and D. The data display form is shown in Table 1:
[0056] Table 1
[0057]
[0058]
[0059] Among them, N in Table 1 A , N B , N C , N D Respectively represent the number of data acquisitions in different periods A, B, C, and D of each cycle; n 1 , n 2 ...n n Indicates the current cycle n 0 The data of the first n cycles of ; x ijkIndicates the data collected for the kth time in the j-th period in the i-th period before the current period; when i=0, it represents the data of the current period; when i=1, it represents the data of the first period before the current period, By analogy, i=n indicates the dat...
PUM
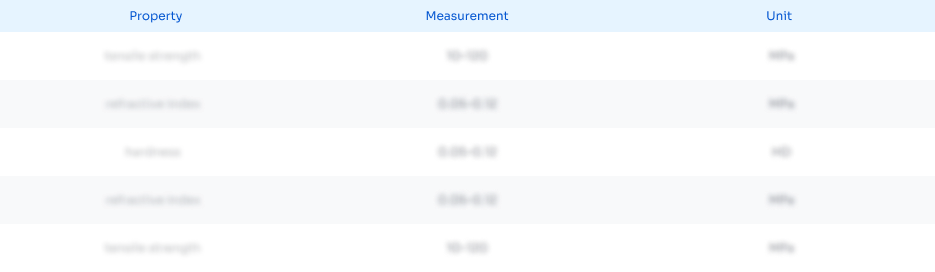
Abstract
Description
Claims
Application Information
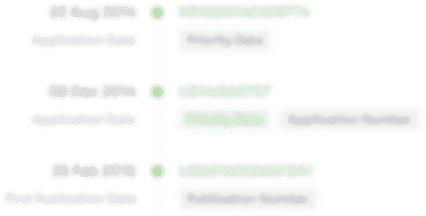
- R&D Engineer
- R&D Manager
- IP Professional
- Industry Leading Data Capabilities
- Powerful AI technology
- Patent DNA Extraction
Browse by: Latest US Patents, China's latest patents, Technical Efficacy Thesaurus, Application Domain, Technology Topic, Popular Technical Reports.
© 2024 PatSnap. All rights reserved.Legal|Privacy policy|Modern Slavery Act Transparency Statement|Sitemap|About US| Contact US: help@patsnap.com