Facet recommendations from sentiment-bearing content
A faceted and emotional technology, applied in speech analysis, natural language translation, natural language data processing, etc., can solve problems such as narrowing of recommendations
- Summary
- Abstract
- Description
- Claims
- Application Information
AI Technical Summary
Problems solved by technology
Method used
Image
Examples
Embodiment Construction
[0017] In the following description of various embodiments of a "faceted recommender" reference is made to the accompanying drawings, which form a part hereof, and in which is shown by way of illustration a specific implementation in which a faceted recommender may be practiced Way. It is to be understood that other embodiments may be utilized and structural changes may be made without departing from the scope thereof.
[0018] It should also be noted that, for the sake of clarity, specific terminology will be resorted to to describe the various embodiments described herein, and that the embodiments are not intended to be limited to the specific terminology so chosen. Moreover, it should be understood that each specific term includes all technical equivalents thereof that operate in a broadly similar manner to accomplish a similar purpose. Reference herein to "one embodiment" or "another embodiment" or "exemplary embodiment" or "alternative embodiment" or similar phrases mean...
PUM
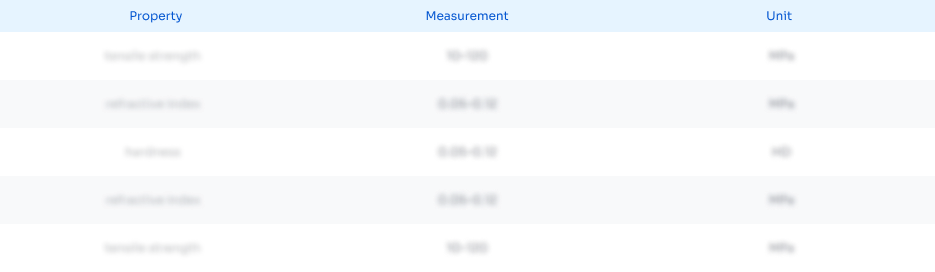
Abstract
Description
Claims
Application Information
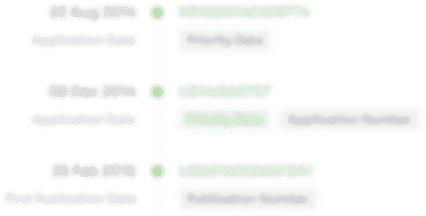
- R&D
- Intellectual Property
- Life Sciences
- Materials
- Tech Scout
- Unparalleled Data Quality
- Higher Quality Content
- 60% Fewer Hallucinations
Browse by: Latest US Patents, China's latest patents, Technical Efficacy Thesaurus, Application Domain, Technology Topic, Popular Technical Reports.
© 2025 PatSnap. All rights reserved.Legal|Privacy policy|Modern Slavery Act Transparency Statement|Sitemap|About US| Contact US: help@patsnap.com