LMD entropy feature and LVQ neural network-based motor imagination electroencephalogram signal identification method
An EEG signal and motor imagery technology, applied in the field of EEG signal recognition and analysis, can solve problems such as endpoint effects, lack of individual adaptive ability, and long time-consuming EMD decomposition
- Summary
- Abstract
- Description
- Claims
- Application Information
AI Technical Summary
Problems solved by technology
Method used
Image
Examples
Embodiment Construction
[0103] Below in conjunction with accompanying drawing and specific embodiment the present invention will be further described:
[0104] In the process of classification and recognition of motor imagery EEG signals, it is first necessary to use electrodes to measure the EEG signal to obtain the EEG signal x(t), then decompose the EEG signal, extract the feature vector, and finally perform recognition to obtain the motor imagery category.
[0105] Such as figure 1 As shown, an EEG signal recognition method based on improved LMD entropy features and LVQ neural network comprehensively applies local mean decomposition algorithm, energy entropy, fuzzy entropy, multi-scale entropy and LVQ neural network. The specific steps of the method are as follows:
[0106] 1. Use signal acquisition equipment to collect EEG signals in the state of motor imagination, including EEG signals for imagining many different sports. Determine the optimal signal response period, signal frequency band and ...
PUM
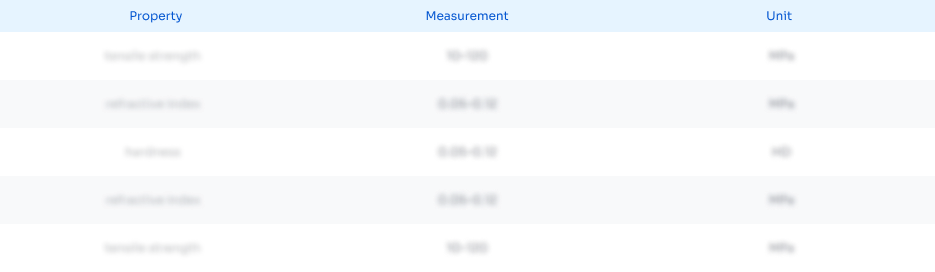
Abstract
Description
Claims
Application Information
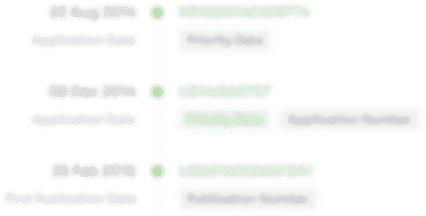
- R&D Engineer
- R&D Manager
- IP Professional
- Industry Leading Data Capabilities
- Powerful AI technology
- Patent DNA Extraction
Browse by: Latest US Patents, China's latest patents, Technical Efficacy Thesaurus, Application Domain, Technology Topic, Popular Technical Reports.
© 2024 PatSnap. All rights reserved.Legal|Privacy policy|Modern Slavery Act Transparency Statement|Sitemap|About US| Contact US: help@patsnap.com