Facial feature recognition method and system based on multi-region feature and metric learning
A technology of metric learning and face features, applied in neural learning methods, character and pattern recognition, instruments, etc., can solve the problems of low face recognition accuracy, slow recognition speed, and high feature dimension
- Summary
- Abstract
- Description
- Claims
- Application Information
AI Technical Summary
Problems solved by technology
Method used
Image
Examples
Embodiment Construction
[0076] The principles of the disclosure will now be described with reference to some example embodiments. It can be understood that these embodiments are described only for the purpose of illustrating and helping those skilled in the art to understand and implement the present disclosure, rather than suggesting any limitation to the scope of the present disclosure. The disclosure described herein may be implemented in various ways other than those described below.
[0077] As used herein, the term "comprising" and its variations may be understood as open-ended terms meaning "including but not limited to". The term "based on" may be understood as "based at least in part on". The term "one embodiment" can be read as "at least one embodiment". The term "another embodiment" may be understood as "at least one other embodiment".
[0078] It can be understood that the following concepts are defined in this embodiment:
[0079] The convolutional neural network is a deep learning a...
PUM
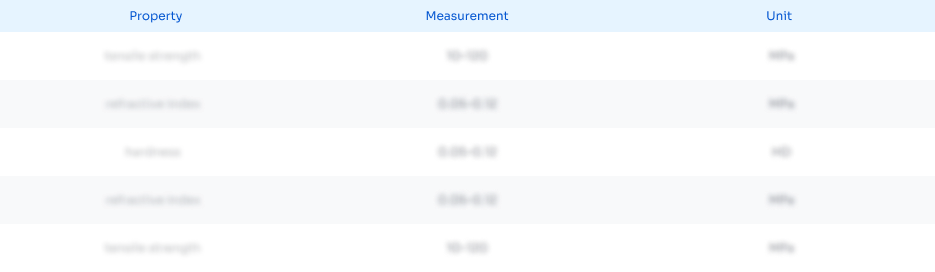
Abstract
Description
Claims
Application Information
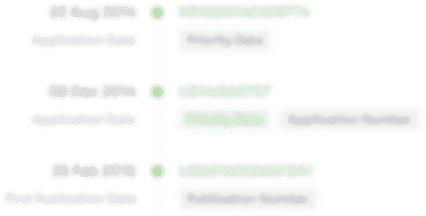
- R&D
- Intellectual Property
- Life Sciences
- Materials
- Tech Scout
- Unparalleled Data Quality
- Higher Quality Content
- 60% Fewer Hallucinations
Browse by: Latest US Patents, China's latest patents, Technical Efficacy Thesaurus, Application Domain, Technology Topic, Popular Technical Reports.
© 2025 PatSnap. All rights reserved.Legal|Privacy policy|Modern Slavery Act Transparency Statement|Sitemap|About US| Contact US: help@patsnap.com