Neural network-based movement recognition method
A neural network and human action recognition technology, applied in neural learning methods, biological neural network models, character and pattern recognition, etc., can solve the problem of less application of convolutional neural networks, and achieve the effect of avoiding negative effects
- Summary
- Abstract
- Description
- Claims
- Application Information
AI Technical Summary
Problems solved by technology
Method used
Image
Examples
Embodiment 1
[0028] The embodiment of the present invention proposes a neural network-based action recognition method, see figure 1 , the action recognition method includes the following steps:
[0029] 101: Train N mutually independent 3D convolutional neural networks based on the video database, and use them as video feature extractors;
[0030] 102: According to the video feature extractor, train a multi-instance learning classifier;
[0031] 103: Input the video to be recognized, extract the features of the video through the trained network, and classify the action through the classifier.
[0032] Wherein, training N mutually independent 3D convolutional neural networks based on the video database in step 101 is used as a video feature extractor specifically as follows:
[0033] Divide each video in the video library into several video clips with a frame length of Fi, and each video clip is used as a training sample for network i to train a 3D convolutional neural network. N independ...
Embodiment 2
[0042] The following combined with specific examples, Figure 2-Figure 4 The scheme in Example 1 is further introduced, see the following description for details:
[0043] 201: Establish a video database, and train N mutually independent 3D convolutional neural networks based on the video database, and use it as a video feature extractor, that is, C3D features;
[0044] Among them, the learning of C3D features is carried out on 3D ConvNets (3D convolutional neural network), and its network structure diagram is as follows figure 2 As shown, all convolution filter sizes are 3*3*3, and the space-time step size is 1. Except for Pool1(1*2*2), all pooling layers have a size of 2*2*2 and a step size of 1. Finally, 4096-dimensional outputs are obtained in the fully connected layers fc6 and fc7, respectively.
[0045] Among them, the video feature extractor needs to train N mutually independent 3D ConvNets, and the training process of each network is the same, see image 3 , takin...
PUM
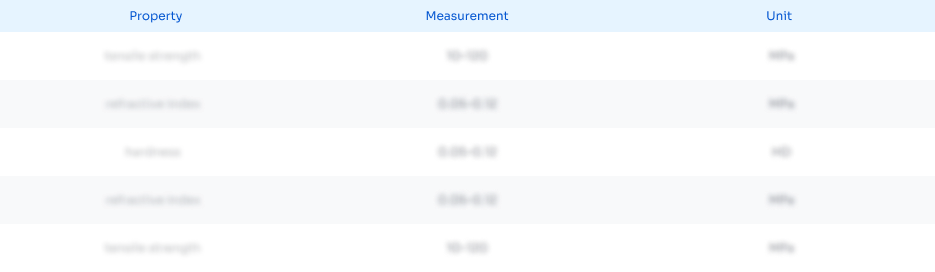
Abstract
Description
Claims
Application Information
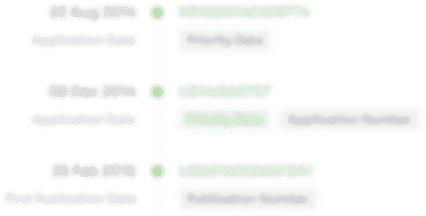
- R&D Engineer
- R&D Manager
- IP Professional
- Industry Leading Data Capabilities
- Powerful AI technology
- Patent DNA Extraction
Browse by: Latest US Patents, China's latest patents, Technical Efficacy Thesaurus, Application Domain, Technology Topic, Popular Technical Reports.
© 2024 PatSnap. All rights reserved.Legal|Privacy policy|Modern Slavery Act Transparency Statement|Sitemap|About US| Contact US: help@patsnap.com