Hyper-parameter determination method for critical convolutional layer of remote-sensing classification convolution neural network
A technology of convolutional neural network and classification method, applied in the field of determination of key convolutional layer hyperparameters of remote sensing classification convolutional neural network, to reduce time and improve classification accuracy
- Summary
- Abstract
- Description
- Claims
- Application Information
AI Technical Summary
Problems solved by technology
Method used
Image
Examples
Embodiment Construction
[0048] The present invention will be further described below in conjunction with specific examples and accompanying drawings.
[0049] The present invention aims to solve the problem of how to determine the convolutional neural network hyperparameters (convolution kernel size and step size) according to different resolution input remote sensing images, and provides a key layer for object-oriented remote sensing classification convolutional neural network based on image input The hyperparameter determination method, and in the convolutional neural network, the first layer of hyperparameters is particularly critical. The purpose of the convolution operation is to extract different features of the input. The first layer of the convolutional layer may only extract some low-level features such as edges. , lines and angles, etc., but it determines whether more layers of the network can iteratively extract more complex features from low-level features. The more complex and reliable fea...
PUM
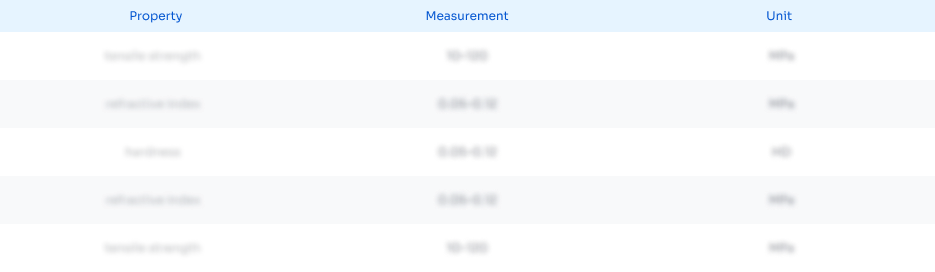
Abstract
Description
Claims
Application Information
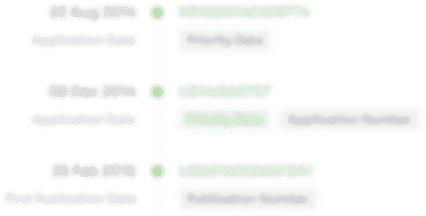
- R&D Engineer
- R&D Manager
- IP Professional
- Industry Leading Data Capabilities
- Powerful AI technology
- Patent DNA Extraction
Browse by: Latest US Patents, China's latest patents, Technical Efficacy Thesaurus, Application Domain, Technology Topic, Popular Technical Reports.
© 2024 PatSnap. All rights reserved.Legal|Privacy policy|Modern Slavery Act Transparency Statement|Sitemap|About US| Contact US: help@patsnap.com