Computer aided pulmonary nodule classification method based on migratable multi-model integration
A computer-aided and classification method technology, applied in computer components, calculations, neural learning methods, etc., can solve problems such as poor accuracy rate, achieve the effect of overcoming low accuracy rate, improving training performance, and increasing accuracy rate
- Summary
- Abstract
- Description
- Claims
- Application Information
AI Technical Summary
Problems solved by technology
Method used
Image
Examples
Embodiment Construction
[0024] The specific steps of the computer-aided pulmonary nodule classification method based on the transferable multi-model integration of the present invention are as follows:
[0025] Step 1. Data preprocessing and data augmentation.
[0026] Since a pulmonary nodule is a spheroid in three-dimensional space, a complete CT image of a pulmonary nodule consists of multiple slices. Based on this phenomenon, the classification problem of 3D pulmonary nodules based on CT images can be transformed into a classification problem in 2D space. Firstly, the original (OA) image sub-blocks that can contain the complete information of pulmonary nodules are extracted on each two-dimensional slice containing pulmonary nodules to describe the global information of pulmonary nodules. To highlight the texture (HVV) and shape (HS) properties of lung nodules, the OA image sub-blocks are next preprocessed. On the one hand, the pixel value of the non-nodule area of the OA image sub-block is se...
PUM
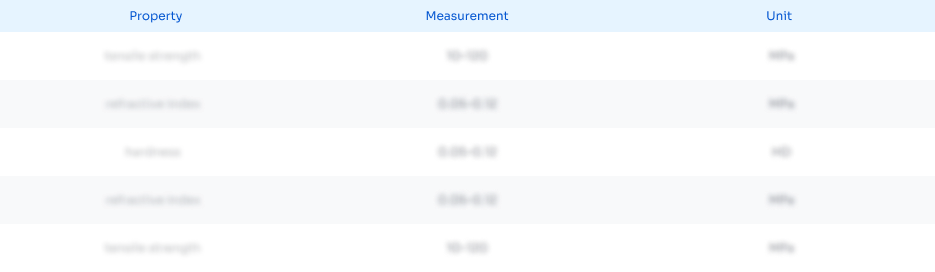
Abstract
Description
Claims
Application Information
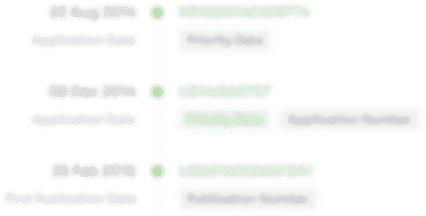
- R&D Engineer
- R&D Manager
- IP Professional
- Industry Leading Data Capabilities
- Powerful AI technology
- Patent DNA Extraction
Browse by: Latest US Patents, China's latest patents, Technical Efficacy Thesaurus, Application Domain, Technology Topic, Popular Technical Reports.
© 2024 PatSnap. All rights reserved.Legal|Privacy policy|Modern Slavery Act Transparency Statement|Sitemap|About US| Contact US: help@patsnap.com