Deep learning-based image high-density population counting method
A deep learning and crowd counting technology, applied in the field of image processing, can solve problems such as perspective distortion, poor effect of crowd counting algorithms, and poor adaptability, and achieve strong generalization ability, easy learning, and good robustness
- Summary
- Abstract
- Description
- Claims
- Application Information
AI Technical Summary
Problems solved by technology
Method used
Image
Examples
Embodiment
[0047] Based on the convolutional neural network theory in deep learning, the present invention proposes a deep and shallow complementary convolutional neural network model to complete the crowd density estimation of a single high-density crowd image. The method flow is as followsfigure 1 Shown:
[0048] First, use the deep learning framework caffe to build a deep and shallow complementary convolutional neural network;
[0049] Then data enhancement is performed on the images in the existing public data sets UCF_CC_50, UCSD, WorldExpo and ShanghaiTech, and finally the image data is enlarged to 192 times;
[0050] After the enhanced image data is processed by Gaussian kernel fuzzy normalization, the real crowd density map is obtained, and the network output estimated density map and real density map are iteratively trained and optimized according to the loss function to optimize the entire network structure;
[0051] The crowd pictures and label pictures are input to the networ...
PUM
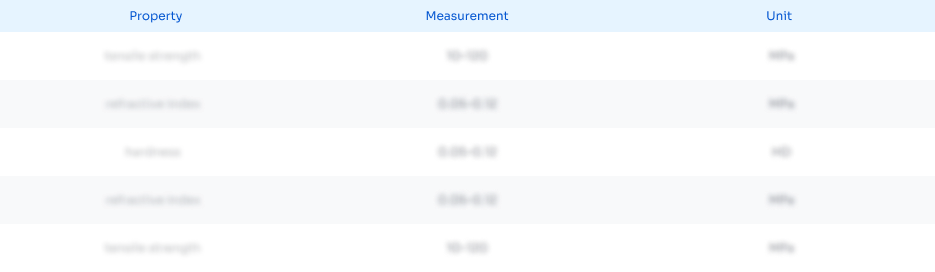
Abstract
Description
Claims
Application Information
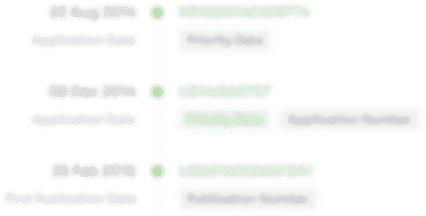
- Generate Ideas
- Intellectual Property
- Life Sciences
- Materials
- Tech Scout
- Unparalleled Data Quality
- Higher Quality Content
- 60% Fewer Hallucinations
Browse by: Latest US Patents, China's latest patents, Technical Efficacy Thesaurus, Application Domain, Technology Topic, Popular Technical Reports.
© 2025 PatSnap. All rights reserved.Legal|Privacy policy|Modern Slavery Act Transparency Statement|Sitemap|About US| Contact US: help@patsnap.com