Sparse representation and dictionary learning-based bearing fault classification method
A sparse representation and dictionary learning technology, applied in character and pattern recognition, instruments, computer parts, etc., can solve the problem of time-consuming solution, achieve the effect of eliminating the feature extraction process, reducing time consumption, and maintaining the quality of the dictionary
- Summary
- Abstract
- Description
- Claims
- Application Information
AI Technical Summary
Problems solved by technology
Method used
Image
Examples
Embodiment Construction
[0053] like figure 1 As shown, a bearing fault classification method based on sparse representation and dictionary learning is to preprocess the collected historical bearing vibration signals, obtain vibration signals under different working conditions, and construct corresponding sub-dictionaries respectively, and then each sub-dictionary Merge into a redundant dictionary; use the sensor to collect the bearing vibration signal online, use the generalized orthogonal matching pursuit algorithm to obtain the sparse coefficient of the signal under the redundant dictionary, and then realize the classification of the vibration signal through the reconstruction error, so as to identify the working state of the bearing ; Specifically, proceed as follows:
[0054] Step 1. From the historical fault vibration signal of the bearing, obtain the training sample set Y={Y corresponding to the K type bearing fault signal 1 ,Y 2 ,...,Y i ,...,Y K}, Y i Indicates the training sample set co...
PUM
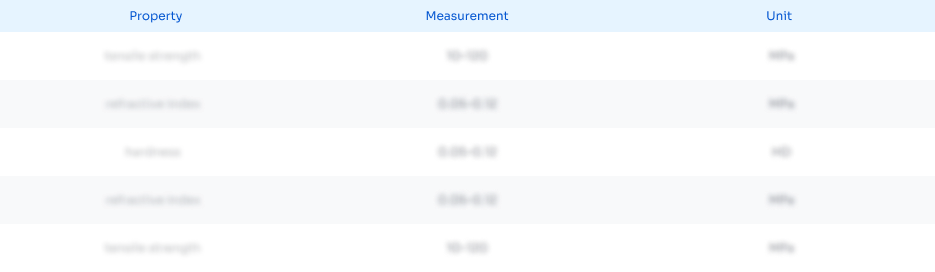
Abstract
Description
Claims
Application Information
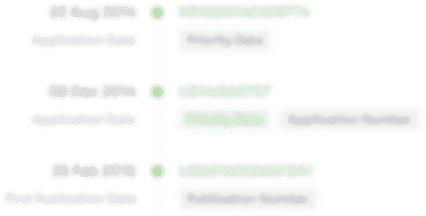
- R&D Engineer
- R&D Manager
- IP Professional
- Industry Leading Data Capabilities
- Powerful AI technology
- Patent DNA Extraction
Browse by: Latest US Patents, China's latest patents, Technical Efficacy Thesaurus, Application Domain, Technology Topic, Popular Technical Reports.
© 2024 PatSnap. All rights reserved.Legal|Privacy policy|Modern Slavery Act Transparency Statement|Sitemap|About US| Contact US: help@patsnap.com