Scene understanding method based on multi-task learning
A multi-task learning and scene understanding technology, applied in neural learning methods, complex mathematical operations, instruments, etc., can solve problems such as unsatisfactory efficiency and prediction accuracy
- Summary
- Abstract
- Description
- Claims
- Application Information
AI Technical Summary
Problems solved by technology
Method used
Image
Examples
Embodiment Construction
[0054] It should be noted that, in the case of no conflict, the embodiments in the present application and the features in the embodiments can be combined with each other. The present invention will be further described in detail below in conjunction with the drawings and specific embodiments.
[0055] figure 1 It is a system framework diagram of a scene understanding method based on multi-task learning in the present invention. It mainly includes multi-task learning with homoscedastic uncertainty, multi-task likelihood function, and scene understanding model.
[0056] Multi-task learning with homoscedastic uncertainty, multi-task learning involves the problem of optimizing a model for multiple objectives; a way to combine multi-objective losses is to perform a weighted linear sum of the losses for each individual task:
[0057]
[0058] But the model performance is not as good as the weight w i The choice of w is very sensitive; at some optimal weights, the joint network...
PUM
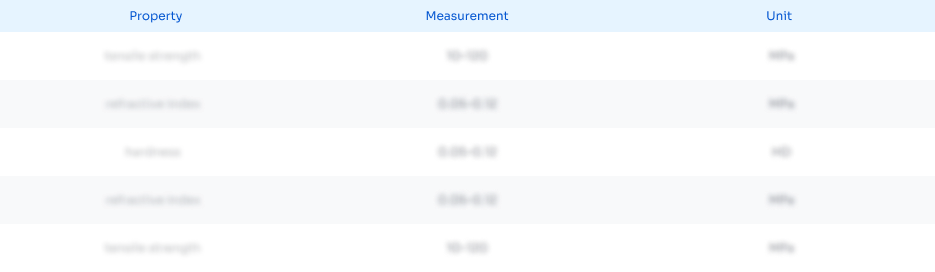
Abstract
Description
Claims
Application Information
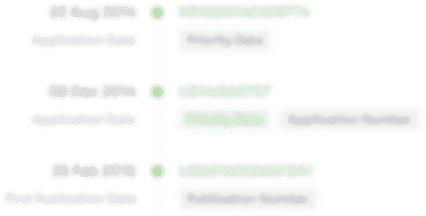
- R&D Engineer
- R&D Manager
- IP Professional
- Industry Leading Data Capabilities
- Powerful AI technology
- Patent DNA Extraction
Browse by: Latest US Patents, China's latest patents, Technical Efficacy Thesaurus, Application Domain, Technology Topic, Popular Technical Reports.
© 2024 PatSnap. All rights reserved.Legal|Privacy policy|Modern Slavery Act Transparency Statement|Sitemap|About US| Contact US: help@patsnap.com