YOLO target detection method using OpenCL
A technology for target detection and testing pictures, applied in the computer field, can solve the problems of lack of generalization ability and low detection accuracy, and achieve the effect of enhancing generalization ability, enhancing portability, and overcoming low target detection accuracy.
- Summary
- Abstract
- Description
- Claims
- Application Information
AI Technical Summary
Problems solved by technology
Method used
Image
Examples
Embodiment Construction
[0034] The present invention will be further described below in conjunction with the accompanying drawings.
[0035] The present invention adopts the OpenCL language, and can be implemented on any NVIDIA GPU device supporting the OpenCL architecture.
[0036] refer to figure 1 , the present invention can be realized through the following steps:
[0037] Step 1, initialize the convolutional neural network.
[0038] Calculate the initial weight value, bias value, and batch normalization scale factor value of the convolutional neural network convolutional layer according to the following formula, and use the calculated three values to initialize the convolutional neural network.
[0039]
[0040]
[0041]
[0042] in, Indicates the nth weight value of the gth channel of the rth layer of the convolutional neural network, ~ means obeying the probability distribution symbol, Indicates the square root operation, π indicates the pi, exp( ) indicates the exponential op...
PUM
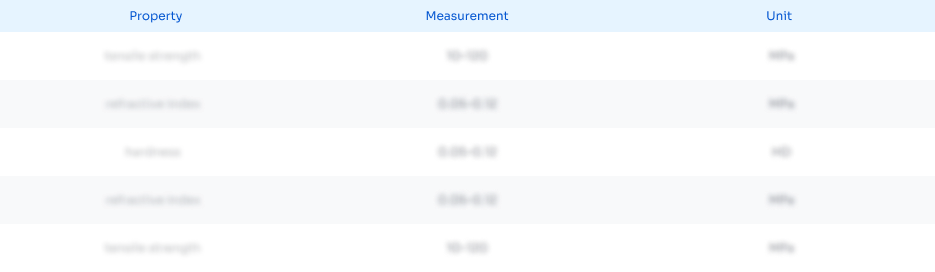
Abstract
Description
Claims
Application Information
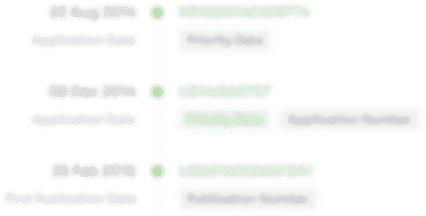
- R&D Engineer
- R&D Manager
- IP Professional
- Industry Leading Data Capabilities
- Powerful AI technology
- Patent DNA Extraction
Browse by: Latest US Patents, China's latest patents, Technical Efficacy Thesaurus, Application Domain, Technology Topic.
© 2024 PatSnap. All rights reserved.Legal|Privacy policy|Modern Slavery Act Transparency Statement|Sitemap