Adaptive semi-supervised density clustering method and system
A density clustering, semi-supervised technique applied in the field of data processing to reduce side effects
- Summary
- Abstract
- Description
- Claims
- Application Information
AI Technical Summary
Problems solved by technology
Method used
Image
Examples
Embodiment Construction
[0049] In order to make the object, technical solution and advantages of the present invention more clear, the present invention will be further described in detail below in conjunction with the examples. It should be understood that the specific embodiments described here are only used to explain the present invention, not to limit the present invention.
[0050] In the self-adaptive semi-supervised density clustering method provided by the present invention, when the data used for clustering has characteristics such as cluster sizes and shapes, the density-based clustering algorithm is a very ideal choice. Unlike the segmentation-based clustering algorithm, it needs to work hard to construct the optimal segmentation of the global data space, such as the DBSCAN algorithm to achieve the optimal region. Furthermore, the density-based semi-supervised learning algorithm can use both Must-Link constraints and Cannot-Link constraints for the data instances that are closest to each ...
PUM
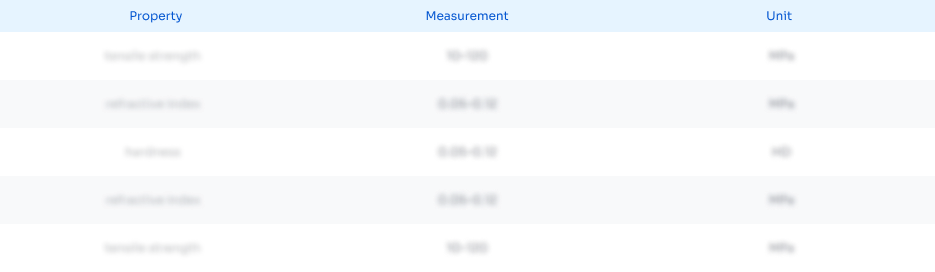
Abstract
Description
Claims
Application Information
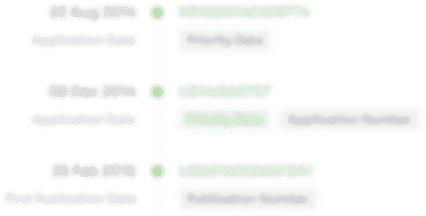
- R&D Engineer
- R&D Manager
- IP Professional
- Industry Leading Data Capabilities
- Powerful AI technology
- Patent DNA Extraction
Browse by: Latest US Patents, China's latest patents, Technical Efficacy Thesaurus, Application Domain, Technology Topic, Popular Technical Reports.
© 2024 PatSnap. All rights reserved.Legal|Privacy policy|Modern Slavery Act Transparency Statement|Sitemap|About US| Contact US: help@patsnap.com