A method of image convolution feature generation based on multi-region cross weights
A multi-area, weighted technology, applied in still image data retrieval, metadata still image retrieval, biological neural network model, etc., can solve the problem of low accuracy, achieve the effect of improving retrieval accuracy and suppressing background noise areas
- Summary
- Abstract
- Description
- Claims
- Application Information
AI Technical Summary
Problems solved by technology
Method used
Image
Examples
Embodiment Construction
[0040] In order to make the object, technical solution and advantages of the present invention clearer, the present invention will be further described in detail below in combination with specific examples and with reference to the accompanying drawings.
[0041] A method for generating image convolution features based on multi-region cross weights, such as figure 1 As shown, it specifically includes the following steps:
[0042] Step 1: Select any convolutional neural network model for classification, and cut off the step of classification in the network (that is, the fully connected layer) to ensure that the network model does not have constraints on the size of the image.
[0043] For the selection of the CNN model, it can be any pre-trained model without any fine-tuning on the retrieved data set. For the CNN model itself, it is necessary to remove the relevant fully connected layers to ensure that the image is input into the CNN network at its original size.
[0044] In ...
PUM
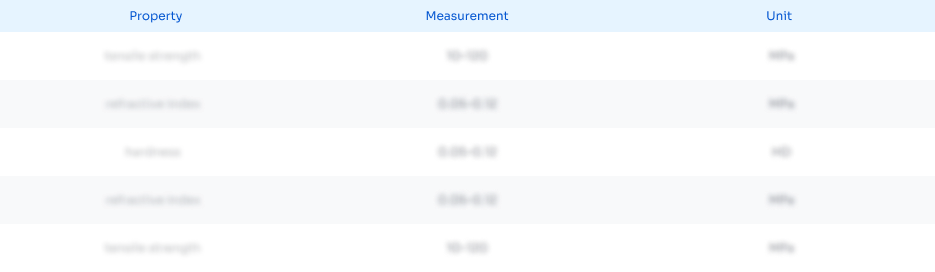
Abstract
Description
Claims
Application Information
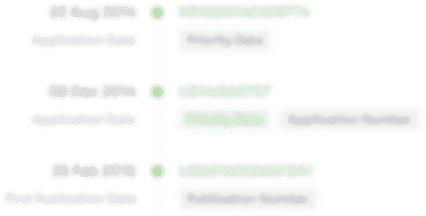
- R&D Engineer
- R&D Manager
- IP Professional
- Industry Leading Data Capabilities
- Powerful AI technology
- Patent DNA Extraction
Browse by: Latest US Patents, China's latest patents, Technical Efficacy Thesaurus, Application Domain, Technology Topic, Popular Technical Reports.
© 2024 PatSnap. All rights reserved.Legal|Privacy policy|Modern Slavery Act Transparency Statement|Sitemap|About US| Contact US: help@patsnap.com