A time-varying feature extraction method for hyperspectral images based on low-rank decomposition and spatial spectral constraints
A hyperspectral image and low-rank decomposition technology, applied in the field of hyperspectral image time-varying feature extraction, can solve the problem of inability to effectively suppress false noise changes, only consider hyperspectral image spectral information, and not make full use of spatial features or spatial spectral characteristics, etc. question
- Summary
- Abstract
- Description
- Claims
- Application Information
AI Technical Summary
Problems solved by technology
Method used
Image
Examples
Embodiment Construction
[0069] Below in conjunction with specific embodiment, further illustrate the present invention. It should be understood that these embodiments are only used to illustrate the present invention and are not intended to limit the scope of the present invention. In addition, it should be understood that after reading the content taught by the present invention, those skilled in the art can make various changes and modifications to the present invention, these etc. Valence forms also fall within the scope defined by the appended claims of the application.
[0070] Embodiments of the present invention relate to a method for extracting time-varying features of hyperspectral images based on low-rank decomposition and spatial spectrum constraints. The algorithm includes the following steps: calculating difference images of time-varying hyperspectral remote sensing images collected at the same place at different times; A low-rank matrix factorization model with space-spectrum constraint...
PUM
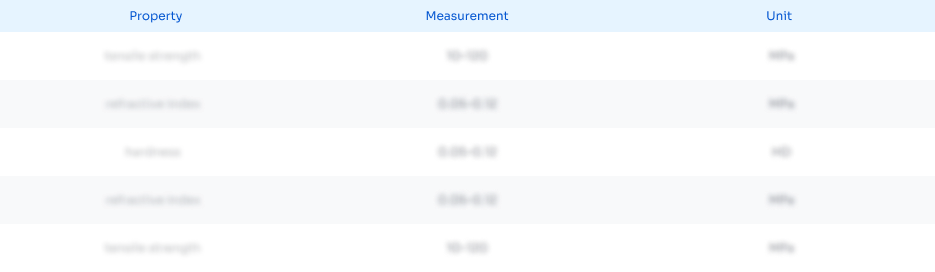
Abstract
Description
Claims
Application Information
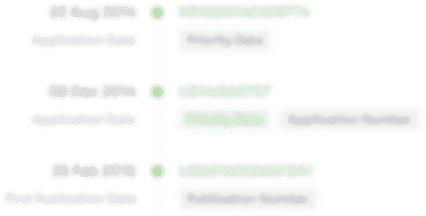
- R&D Engineer
- R&D Manager
- IP Professional
- Industry Leading Data Capabilities
- Powerful AI technology
- Patent DNA Extraction
Browse by: Latest US Patents, China's latest patents, Technical Efficacy Thesaurus, Application Domain, Technology Topic, Popular Technical Reports.
© 2024 PatSnap. All rights reserved.Legal|Privacy policy|Modern Slavery Act Transparency Statement|Sitemap|About US| Contact US: help@patsnap.com