Variational automatic encoder-based zero-sample image classification method
An autoencoder and sample image technology, applied to neural learning methods, instruments, computer components, etc., can solve problems such as labor-intensive and lack of labeled data
- Summary
- Abstract
- Description
- Claims
- Application Information
AI Technical Summary
Problems solved by technology
Method used
Image
Examples
Embodiment Construction
[0041] Given a set of visible class samples S={(x i ,z i ,y i ),i=1,...,n}, is the visual feature of the visible class sample, is the semantic feature of visible class samples, is the category of visible class samples, and n is the number of visible class samples. The purpose of zero-shot classification is to classify the visual features of a given unseen class sample j=1,...,m (m is the number of unseen class samples) and semantic features of all unseen class categories (t is the number of categories of unseen classes), predicting the category of unseen class samples j=1,...,m, where
[0042] The current method to solve the zero-shot image classification problem mainly includes the following three steps:
[0043] 1) Use training samples to train visual space to semantic space map f: or semantic space to visual space map g: Semantic embedding model of ;
[0044] 2) Use the learned model to map samples of unknown categories to semantic space, or map ...
PUM
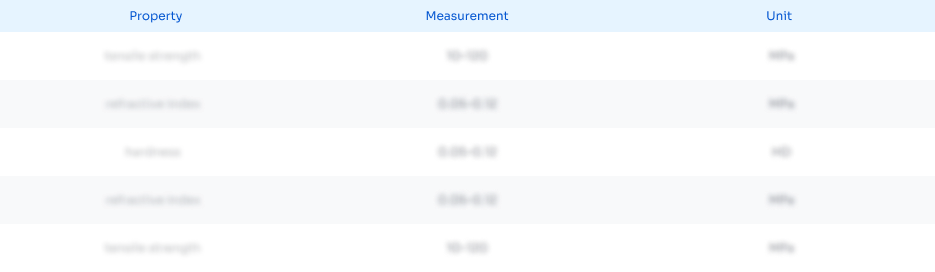
Abstract
Description
Claims
Application Information
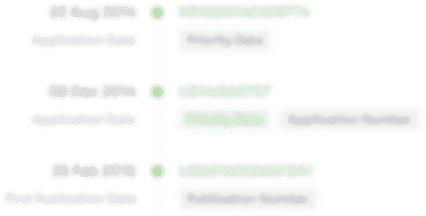
- R&D Engineer
- R&D Manager
- IP Professional
- Industry Leading Data Capabilities
- Powerful AI technology
- Patent DNA Extraction
Browse by: Latest US Patents, China's latest patents, Technical Efficacy Thesaurus, Application Domain, Technology Topic, Popular Technical Reports.
© 2024 PatSnap. All rights reserved.Legal|Privacy policy|Modern Slavery Act Transparency Statement|Sitemap|About US| Contact US: help@patsnap.com