Image semantic segmentation method based on confrontation training
A semantic segmentation and image technology, which is applied in the field of computer vision, can solve the problems of ignoring the local features of the picture, and cannot realize the meaningful fusion of the global information and local information of the image, so as to avoid the process of initializing parameters, increase interpretability, and train stably Effect
- Summary
- Abstract
- Description
- Claims
- Application Information
AI Technical Summary
Problems solved by technology
Method used
Image
Examples
Embodiment 1
[0044] combined with figure 1 , the image semantic segmentation method based on adversarial training of the present embodiment, comprises the following steps:
[0045] Step 1: Input the original image to the convolutional neural network (i.e., the generation network G) for forward transfer to obtain a low-resolution segmented image;
[0046] Specifically: set the size of the original image as H×W×3, input the original image to the convolutional neural network (that is, the generation network G) for convolution pooling operation, and obtain the first downsampling feature layer with a size of H / the s 1 ×W / s 1 ×C down1 , and then perform convolution and pooling operations on the first downsampled feature layer again to obtain the second downsampled feature layer with a size of H / (s 1 ×s 2 )×W / (s 1 ×s 2 )×C down2 , repeating this process, the third downsampling feature layer, the fourth downsampling feature layer, etc. can be obtained in turn. For the sake of simplicity, ...
PUM
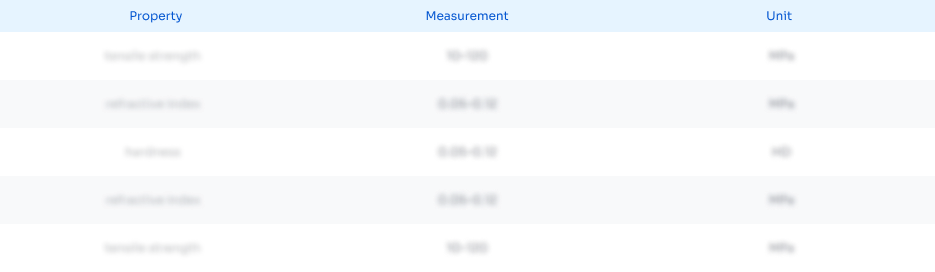
Abstract
Description
Claims
Application Information
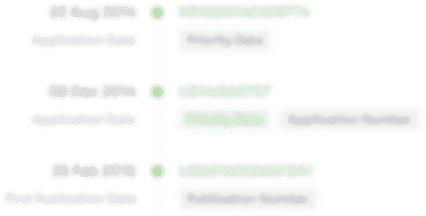
- R&D Engineer
- R&D Manager
- IP Professional
- Industry Leading Data Capabilities
- Powerful AI technology
- Patent DNA Extraction
Browse by: Latest US Patents, China's latest patents, Technical Efficacy Thesaurus, Application Domain, Technology Topic, Popular Technical Reports.
© 2024 PatSnap. All rights reserved.Legal|Privacy policy|Modern Slavery Act Transparency Statement|Sitemap|About US| Contact US: help@patsnap.com