Feature selection method based on group sparse norm and local learning
A feature selection method and local learning technology, applied in computer parts, character and pattern recognition, instruments, etc., can solve problems such as ignoring the structure of data groups, and achieve good robustness and accuracy
- Summary
- Abstract
- Description
- Claims
- Application Information
AI Technical Summary
Problems solved by technology
Method used
Image
Examples
Embodiment Construction
[0024] With reference to accompanying drawing, further illustrate the present invention:
[0025] S1: Use a local learning method to infer the label information of the missing label data in different classes;
[0026] S2: Fit the label information obtained by S1, and use the group sparse specification;
[0027] S3: According to the regression coefficient obtained in S2, calculate the score of each feature, and select a set of features with the highest score.
[0028] Further, the method of local learning of S1 inferring missing label information is as follows:
[0029] (2.1) Organize the data set into an N×M matrix X, N is the number of data, M is the number of features; mark the label information matrix Y, the nth row and the cth column of the matrix Y indicate whether the data n is in the class c ;
[0030] (2.2) Construct the k-nearest neighbor graph of the data set and the kernel matrix K of matrix X; where the k-nearest neighbor graph is used to obtain the k nearest ne...
PUM
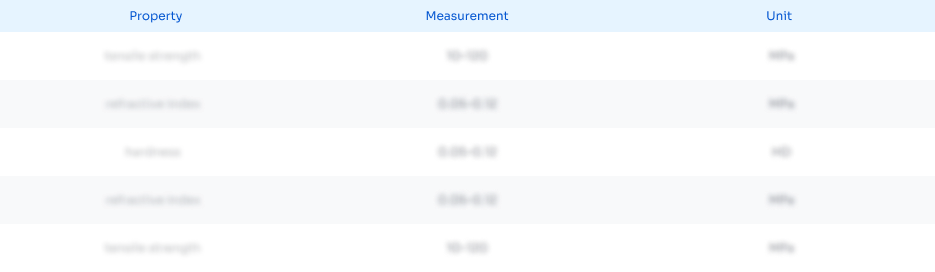
Abstract
Description
Claims
Application Information
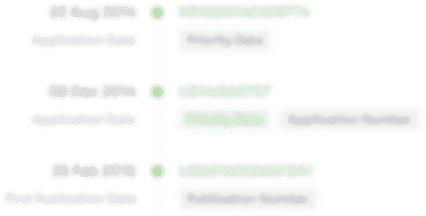
- R&D
- Intellectual Property
- Life Sciences
- Materials
- Tech Scout
- Unparalleled Data Quality
- Higher Quality Content
- 60% Fewer Hallucinations
Browse by: Latest US Patents, China's latest patents, Technical Efficacy Thesaurus, Application Domain, Technology Topic, Popular Technical Reports.
© 2025 PatSnap. All rights reserved.Legal|Privacy policy|Modern Slavery Act Transparency Statement|Sitemap|About US| Contact US: help@patsnap.com