Division and identification method and device based on dense network image
A dense network and recognition method technology, applied in image analysis, image coding, image data processing, etc., can solve problems such as low resolution, no pooling operation, and inability to obtain high-resolution segmentation results
- Summary
- Abstract
- Description
- Claims
- Application Information
AI Technical Summary
Problems solved by technology
Method used
Image
Examples
Embodiment 1
[0053] see figure 1 and figure 2 The segmentation and recognition method based on the dense network image proposed in this embodiment specifically includes the following steps:
[0054] Step S101: Use the convolutional layer as a feature extractor to capture the hierarchical features of dense network images, and input the obtained multi-scale feature maps into the encoding module. At the same time, use skip connections to combine the feature maps of different scales with the encoding module. The feature maps corresponding to the scales are connected to obtain multiple feature maps.
[0055] Step S102: use the encoding module to down-sample the feature map and extract semantic features, use the decoding module to up-sample the feature map and recover detailed information, and obtain a multi-scale feature map.
[0056] Step S103: Perform a chained residual pooling operation on the output feature map obtained by the dense deconvolution operation, wherein the output feature map...
Embodiment 2
[0075] see image 3 , Figure 4 and Figure 5 , the present embodiment provides a segmentation based on a dense network image, and the recognition device includes: a convolution module 1 is used to use a convolutional layer as a feature extractor to capture the hierarchical features of a dense network image, and input the obtained multi-scale feature map to the encoding module, and at the same time, use skip connections to connect feature maps of different scales with feature maps of corresponding scales in the encoding module to obtain multiple feature maps. The multi-scale feature map acquisition module 2 is used to use the encoding module to download the feature maps Sampling and semantic feature extraction, use the decoding module to upsample the feature map and restore detailed information to obtain multi-scale feature maps, the pooling operation module 3 is used to perform chain residual pooling on the output feature map obtained by the dense deconvolution operation op...
PUM
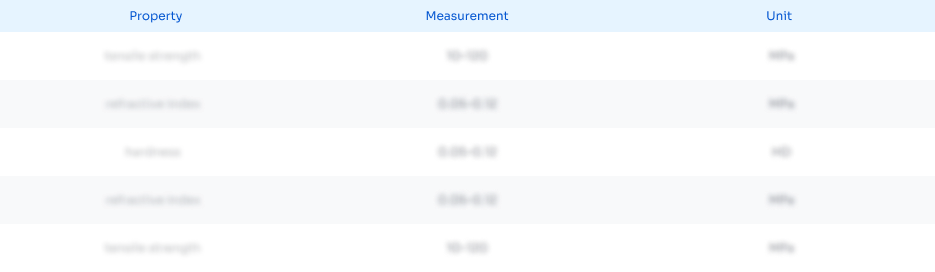
Abstract
Description
Claims
Application Information
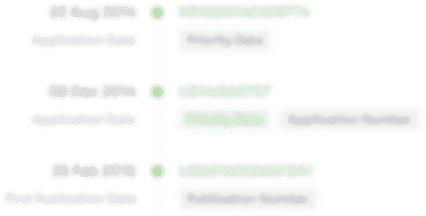
- R&D
- Intellectual Property
- Life Sciences
- Materials
- Tech Scout
- Unparalleled Data Quality
- Higher Quality Content
- 60% Fewer Hallucinations
Browse by: Latest US Patents, China's latest patents, Technical Efficacy Thesaurus, Application Domain, Technology Topic, Popular Technical Reports.
© 2025 PatSnap. All rights reserved.Legal|Privacy policy|Modern Slavery Act Transparency Statement|Sitemap|About US| Contact US: help@patsnap.com