Audio classification method based on convolution neural network and random forest
A convolutional neural network and random forest technology, applied in biological neural network models, speech analysis, neural architecture, etc., can solve the problem of weak generalization ability of a single classifier, and achieve strong feature extraction ability, high accuracy and high accuracy. Recall, the effect of simplifying complex processes
- Summary
- Abstract
- Description
- Claims
- Application Information
AI Technical Summary
Problems solved by technology
Method used
Image
Examples
Embodiment 1
[0041] Embodiment 1 is an example of the present invention, using "GTZAN Genre Collection" as a data set, adopting nine kinds of audio files of different genres as a training set and a test set, nine kinds of categories are: blues, C1assical, Country, Disco, Jazz, Metal, Pop, Reggae and Rock.
[0042] 1. Divide the audio file into 6 segments of equal length, and each segment corresponds to the same tag. Framing, windowing, and Fourier transform each segment of audio to obtain its spectrogram. attached figure 2 Shown is the acquired spectrum. Read in the spectrogram and convert it to grayscale. Then adjust the size of each picture to 248*248. Finally, save the pixel values of the adjusted image to an array as a sample in the convolutional neural network dataset. After the above operations, the data set D (5400, 248, 248) is obtained, which means that there are 5400 spectrograms, and the width of each spectrogram is 248 and the height is 248. The data set is divided int...
PUM
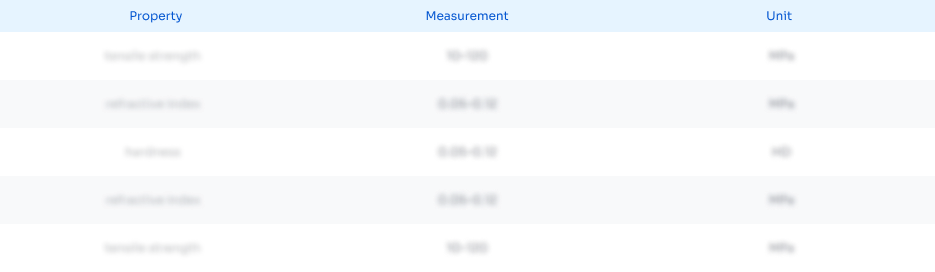
Abstract
Description
Claims
Application Information
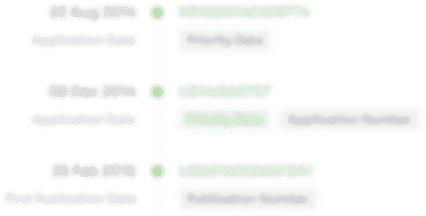
- R&D Engineer
- R&D Manager
- IP Professional
- Industry Leading Data Capabilities
- Powerful AI technology
- Patent DNA Extraction
Browse by: Latest US Patents, China's latest patents, Technical Efficacy Thesaurus, Application Domain, Technology Topic, Popular Technical Reports.
© 2024 PatSnap. All rights reserved.Legal|Privacy policy|Modern Slavery Act Transparency Statement|Sitemap|About US| Contact US: help@patsnap.com