Model training method and device, electronic equipment
A technology of model training and training samples, applied in the computer field, can solve problems such as inaccurate prediction results, achieve the effect of improving the accuracy of prediction results and avoiding uneven distribution of training data
- Summary
- Abstract
- Description
- Claims
- Application Information
AI Technical Summary
Problems solved by technology
Method used
Image
Examples
Embodiment 1
[0026] A model training method disclosed in this embodiment, such as figure 1 As shown, the method includes: step 110 to step 140.
[0027] Step 110, according to the training samples, obtain at least a subset of training samples.
[0028] The training samples used to train the model usually include: sample labels and sample features of preset dimensions. The preset dimensions are selected according to different application scenarios of the source data and the model to be trained. Taking the prediction of the user's purchase rate as an example, the possible preset dimensions include: user gender, age, occupation, place of residence, commodity category, price, number of purchases and other characteristics. The larger the number of training samples, the more accurate the prediction results of the trained model will be. During the specific implementation of the present application, the training samples are first sampled to obtain multiple sub-training samples, which are respec...
Embodiment 2
[0038] A model training method disclosed in this embodiment, such as figure 2 As shown, the method includes: Step 210 to Step 270.
[0039] Step 210: Obtain at least a subset of training samples according to the training samples.
[0040]The larger the number of training samples, the more accurate the prediction results of the trained model will be. During the specific implementation of the present application, the training samples are first sampled to obtain multiple sub-training samples, which are respectively used to train different machine learning models. During specific implementation, the obtaining at least one sub-training sample according to the training sample includes: performing random sampling on the training sample to obtain at least one sub-training sample; and performing feature sampling on each sub-training sample. Assuming that there are 10,000 training samples in total, to obtain 10 sub-training samples, 80% of the total training samples can be randomly s...
Embodiment 3
[0077] A model training device disclosed in this embodiment, such as Figure 4 As shown, the device includes:
[0078] A sampling module 410, configured to obtain at least one sub-training sample according to the training sample;
[0079] The single-model training and prediction module 420 is used to respectively train a plurality of machine learning models corresponding to each of the sub-training samples according to each of the sub-training samples, and obtain a corresponding machine learning model for each of the sub-training samples. predicted value;
[0080] A sample feature fusion module 430, configured to determine a fusion training sample according to the predicted value;
[0081] The target machine model training module 440 is configured to train the target machine learning model according to the fused training samples determined by the sample feature fusion module 430 .
[0082] optional, such as Figure 5 As shown, the target machine model training module 440 f...
PUM
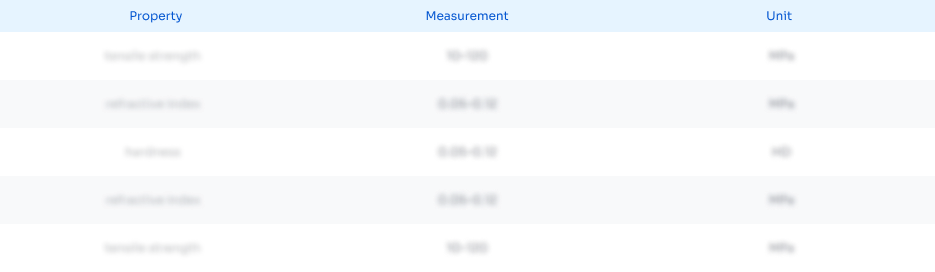
Abstract
Description
Claims
Application Information
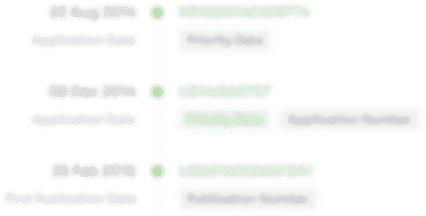
- Generate Ideas
- Intellectual Property
- Life Sciences
- Materials
- Tech Scout
- Unparalleled Data Quality
- Higher Quality Content
- 60% Fewer Hallucinations
Browse by: Latest US Patents, China's latest patents, Technical Efficacy Thesaurus, Application Domain, Technology Topic, Popular Technical Reports.
© 2025 PatSnap. All rights reserved.Legal|Privacy policy|Modern Slavery Act Transparency Statement|Sitemap|About US| Contact US: help@patsnap.com