Image text matching model training method, bidirectional search method and related device
A technology for matching models and image samples, applied in the field of artificial intelligence, can solve problems such as the inability to comprehensively measure the matching degree of images and texts, and achieve the effect of accurate matching, accurate search results, and comprehensive matching.
- Summary
- Abstract
- Description
- Claims
- Application Information
AI Technical Summary
Problems solved by technology
Method used
Image
Examples
Embodiment 1
[0055] refer to figure 2 , is a flow chart of a training method for an image-text matching model provided in Embodiment 1 of the present application, including the following steps:
[0056] Step 201: Extract global representation and local representation of image samples.
[0057] Step 202: Extract global and local representations of text samples.
[0058] It should be noted that the execution sequence of step 201 and step 202 is not limited.
[0059] Step 203: According to the extracted global representation and local representation, train a pre-built matching model, so that the matching model can determine the matching degree between the image and the text based on the global representation and the local representation;
[0060] Among them, the matching model maps the respective global representations of image samples and text samples to the specified semantic space, and calculates the similarity of global representations between heterogeneous sample pairs composed of ima...
Embodiment 2
[0109] Such as Figure 7 As shown, it is a schematic flow chart of a specific embodiment of the training method of the image-text matching model provided in the embodiment of the present application. The method includes the following steps:
[0110] Step 701: Extract the global representation of the image sample based on the global image representation CNN.
[0111] Step 702: Divide the image sample into a specified number of image blocks, and calculate the probability that the image block contains the image information of the specified category for each image block based on the local image CNN; and select each specified category in the specified number of image blocks The maximum probability of the image information of each specified category constitutes the local representation of the image sample.
[0112] Step 703: segment the text sample; for each word segment, determine the vector of the word segment, wherein the vectors of different word segments have the same length; ...
Embodiment 3
[0122] Such as Figure 8 As shown, it is a flow chart of the image-text bidirectional search method based on the matching model in Embodiment 1, including the following steps:
[0123] Step 801: Receive a reference sample, where the reference sample is text or an image.
[0124] Step 802: Extract global representation and local representation of the reference sample.
[0125] Step 803: Input the global representation and locality of the reference sample into the matching model, so that the matching model can calculate the matching degree between the reference sample and the corresponding material; wherein, if the reference sample is text, then the corresponding material is an image; if the reference sample is image, the corresponding material is text; the matching model can determine the matching degree between the image and the text based on the global representation and the local representation.
[0126] Wherein, a material library may be established, and a matching degree...
PUM
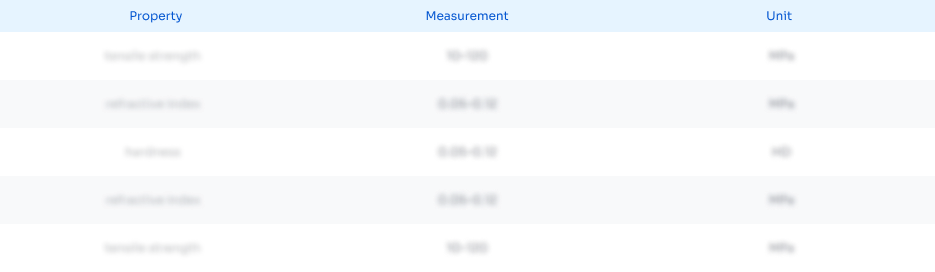
Abstract
Description
Claims
Application Information
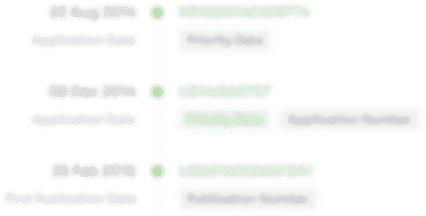
- R&D
- Intellectual Property
- Life Sciences
- Materials
- Tech Scout
- Unparalleled Data Quality
- Higher Quality Content
- 60% Fewer Hallucinations
Browse by: Latest US Patents, China's latest patents, Technical Efficacy Thesaurus, Application Domain, Technology Topic, Popular Technical Reports.
© 2025 PatSnap. All rights reserved.Legal|Privacy policy|Modern Slavery Act Transparency Statement|Sitemap|About US| Contact US: help@patsnap.com