Mobile Nets-based method for optimizing multi-scale learning network
A learning network, multi-scale technology, applied in the field of deep neural network, can solve problems such as unoptimistic accuracy
- Summary
- Abstract
- Description
- Claims
- Application Information
AI Technical Summary
Problems solved by technology
Method used
Image
Examples
Embodiment Construction
[0038] The technical solutions of the present invention will be further described below in conjunction with the accompanying drawings and embodiments.
[0039] The present invention is an optimized neural network based on the MobileNets network, and its steps include:
[0040] Step 1, as shown in Table 1, where 3*3*3 and 1*1*3 represent the depth convolution kernel of 3*3 size and the point convolution kernel of 1*1 size respectively. The network constructed by the present invention can be divided into 4 parts, the first 3 parts are the same separable convolutional layer, each separable convolutional layer is connected to batchnorm and ReLU, and then connected to the pooling layer, the fourth part is fully connected Layer and output layer, now take the convolution process of the first part as an example to introduce, the specific network structure see image 3 . The hidden layer structure of the first part is divided into 3 groups. The first group performs convolution operat...
PUM
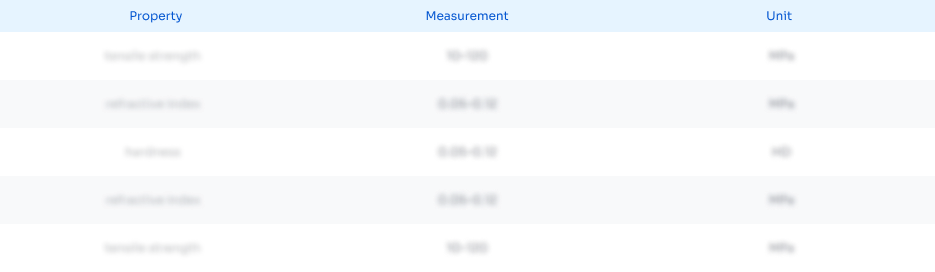
Abstract
Description
Claims
Application Information
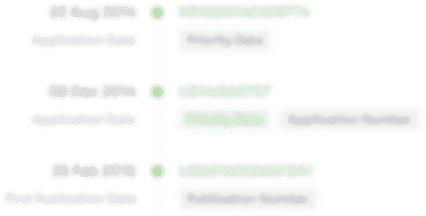
- R&D Engineer
- R&D Manager
- IP Professional
- Industry Leading Data Capabilities
- Powerful AI technology
- Patent DNA Extraction
Browse by: Latest US Patents, China's latest patents, Technical Efficacy Thesaurus, Application Domain, Technology Topic, Popular Technical Reports.
© 2024 PatSnap. All rights reserved.Legal|Privacy policy|Modern Slavery Act Transparency Statement|Sitemap|About US| Contact US: help@patsnap.com