Bearing fault diagnosis method based on average multi-granularity decision rough set and NNBC
A fault diagnosis, multi-granularity technology, applied in mechanical bearing testing, measuring devices, instruments, etc., can solve problems such as inappropriate selection of constraints, improve clustering effect, reduce computational complexity, and reduce the number of input variables. Effect
- Summary
- Abstract
- Description
- Claims
- Application Information
AI Technical Summary
Problems solved by technology
Method used
Image
Examples
specific Embodiment approach 1
[0033] Specific implementation mode one: combine figure 1 Illustrate this embodiment, based on average multi-granularity decision-making rough set and NNBC bearing fault diagnosis method, it is characterized in that the method comprises the following steps:
[0034] Step 1, extracting the fault diagnosis features of rolling bearings in the training samples to construct a fault symptom attribute set;
[0035] Step 2. Using the attribute reduction algorithm based on the average multi-granularity decision-making rough set to reduce the dimensionality of the symptom attribute set in the training sample;
[0036] Step 3: Construct NNBC based on the reduced training samples, and use the NNBC-based pattern recognition algorithm to judge the rolling bearing status of the samples to be diagnosed.
specific Embodiment approach 2
[0037] Specific embodiment two: the difference between this embodiment and specific embodiment one is: the attribute reduction algorithm based on the average multi-granularity decision-making rough set in the step two, combined with figure 2 Describe this implementation mode:
[0038] Step 21. According to the risk price λ p,q (p,q=1,2,3), calculate the threshold α; where
[0039] Step 22. Calculate the classification quality S of the symptom attribute set C with respect to the decision attribute D C (D);
[0040] Step two and three, in turn for each symptom attribute c i ,i=1,2,...,n perform the following operations;
[0041] Step 24: Calculate symptom attribute c i In the symptom attribute set C, the importance Sig(c i ,C,D);
[0042] Step 25, if the symptom attribute c i The importance of Sig(c i ,C,D)=0, then the symptom attribute c i is redundant, otherwise essential;
[0043] Step 26. Repeat steps 24 and 25 for other symptom attributes until the last sympto...
specific Embodiment approach 3
[0045] Embodiment 3: The difference between this embodiment and Embodiment 1 or 2 is: the classification quality S of the symptom attribute set C with respect to the decision attribute D in the step 22 C (D) is defined as follows:
[0046] For the information system S=(U,A=C∪D,V,f), U is the instance set, A is the attribute set, C is the symptom attribute set, D is the decision attribute set, V is the range of A, f is Mapping function, let B={B 1 ,B 2 ,...,B m} are m attribute subsets of symptom attribute set C, then the classification quality of symptom attribute set C with respect to decision attribute D is:
[0047]
[0048] in, is the lower approximate set of the average multi-granularity decision rough set of set X about B, and α is the threshold.
PUM
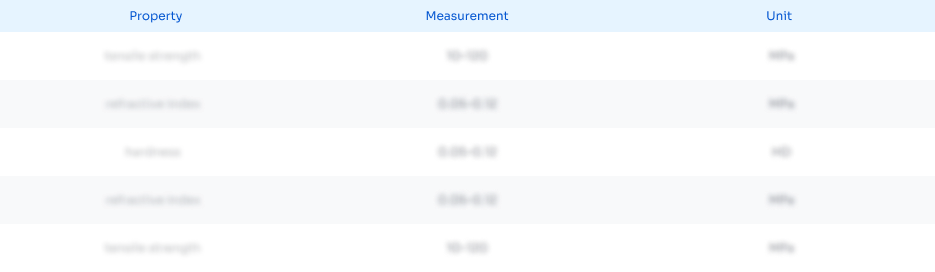
Abstract
Description
Claims
Application Information
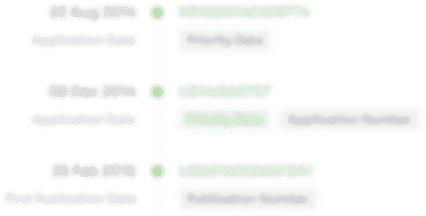
- R&D Engineer
- R&D Manager
- IP Professional
- Industry Leading Data Capabilities
- Powerful AI technology
- Patent DNA Extraction
Browse by: Latest US Patents, China's latest patents, Technical Efficacy Thesaurus, Application Domain, Technology Topic, Popular Technical Reports.
© 2024 PatSnap. All rights reserved.Legal|Privacy policy|Modern Slavery Act Transparency Statement|Sitemap|About US| Contact US: help@patsnap.com