A point cloud semantic map construction method based on deep learning and lidar
A technology of lidar and semantic map, applied in neural architecture, 3D modeling, image data processing, etc., can solve the problems of inapplicability, cumbersome process, complicated calibration process, etc., and achieve accurate effect, strong robustness, and simple process Effect
- Summary
- Abstract
- Description
- Claims
- Application Information
AI Technical Summary
Problems solved by technology
Method used
Image
Examples
Embodiment Construction
[0034] The accompanying drawings are for illustrative purposes only, and should not be construed as limitations on this patent; in order to better illustrate this embodiment, certain components in the accompanying drawings will be omitted, enlarged or reduced, and do not represent the size of the actual product; for those skilled in the art It is understandable that some well-known structures and descriptions thereof may be omitted in the drawings. The positional relationship described in the drawings is for illustrative purposes only, and should not be construed as a limitation on this patent.
[0035]The purpose of the present invention is to provide a point cloud semantic map construction method based on deep learning and laser radar. The present invention uses a lightweight deep convolutional network specially designed for the semantic segmentation of laser radar point clouds to carry out real-time semantic annotation on the preprocessed laser radar point cloud to obtain t...
PUM
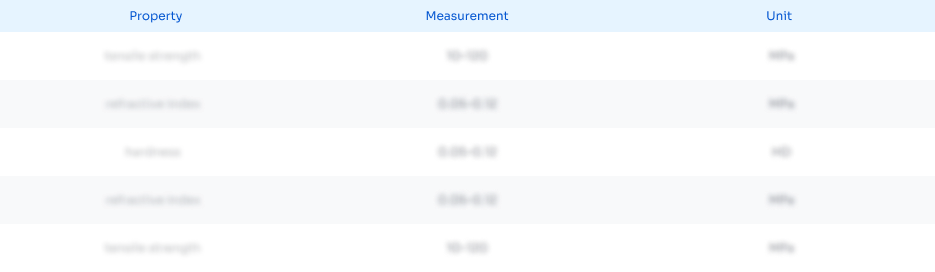
Abstract
Description
Claims
Application Information
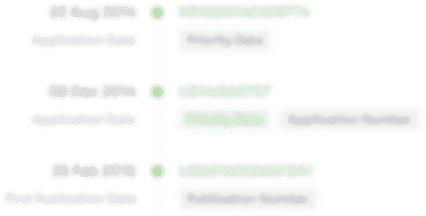
- R&D Engineer
- R&D Manager
- IP Professional
- Industry Leading Data Capabilities
- Powerful AI technology
- Patent DNA Extraction
Browse by: Latest US Patents, China's latest patents, Technical Efficacy Thesaurus, Application Domain, Technology Topic.
© 2024 PatSnap. All rights reserved.Legal|Privacy policy|Modern Slavery Act Transparency Statement|Sitemap