An Adaptive Fuzzy Neural Network Gear Remaining Life Prediction Method
An adaptive fuzzy, neural network technology, applied in biological neural network models, machine gear/transmission mechanism testing, neural architecture, etc., can solve the problems of casualties, downtime, and high vibration frequency of gears
- Summary
- Abstract
- Description
- Claims
- Application Information
AI Technical Summary
Problems solved by technology
Method used
Image
Examples
Embodiment Construction
[0048] A kind of embodiment of the present invention is described further below in conjunction with accompanying drawing:
[0049] In the embodiment of the present invention, the method for predicting the remaining life of gears based on fuzzy neural network, the method flow chart is as follows figure 1 shown, including the following steps:
[0050] Step 1. Carry out fatigue tests on gears to obtain real-time monitoring data that characterizes gear degradation:
[0051] The gear fatigue life test adopts a power flow closed test bench. The center distance of the test bench is 150mm, and the motor speed is 1200r / min. During the test, the vibration of the box, oil temperature and noise are monitored. The material used in the test is alloy steel. , hard tooth surface gear with a tooth surface hardness of 58-61HRC, the surface treatment is carburized and quenched, and the front and back sides are staggered and overlapped. The main test box gear modulus is m=3, the number of teeth ...
PUM
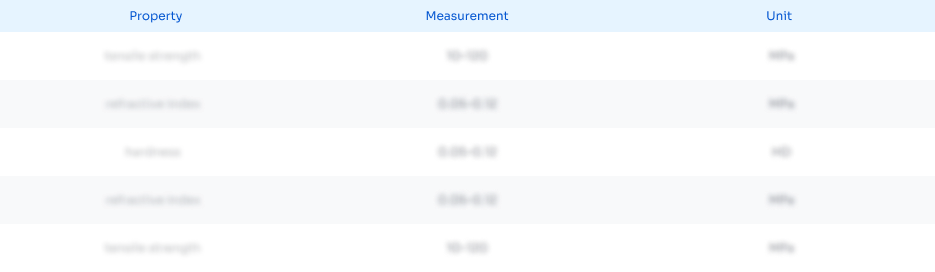
Abstract
Description
Claims
Application Information
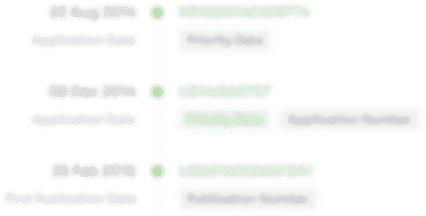
- R&D Engineer
- R&D Manager
- IP Professional
- Industry Leading Data Capabilities
- Powerful AI technology
- Patent DNA Extraction
Browse by: Latest US Patents, China's latest patents, Technical Efficacy Thesaurus, Application Domain, Technology Topic, Popular Technical Reports.
© 2024 PatSnap. All rights reserved.Legal|Privacy policy|Modern Slavery Act Transparency Statement|Sitemap|About US| Contact US: help@patsnap.com