Cascaded residual error neural network-based image denoising method
A neural network and neural network model technology, applied in the field of computer vision and digital image processing, can solve the problems of image noise and resolution that are not robust, lack of practical application value, inaccurate models, etc., to avoid gradient explosion, The effect of improving efficiency and quality and enhancing learning ability
- Summary
- Abstract
- Description
- Claims
- Application Information
AI Technical Summary
Problems solved by technology
Method used
Image
Examples
Embodiment Construction
[0031] The present invention will be further described below with reference to the accompanying drawings and in combination with preferred embodiments.
[0032] The image denoising method based on the cascaded residual neural network of the present invention introduces a convolutional layer, an activation layer and a unit skip connection unit, and obtains good features on the basis of the learning ability of the convolutional layer and the screening ability of the activation layer , directly connect the input and output through the unit jump connection unit, retain more detailed information of the input image, enhance the feature extraction of the neural network model, and increase the convergence speed of the neural network model training process; thereby greatly enhancing the learning of the neural network Ability to accurately learn the mapping from noisy images to clean images to establish an input-to-output mapping, and finally predict and estimate clean images through the...
PUM
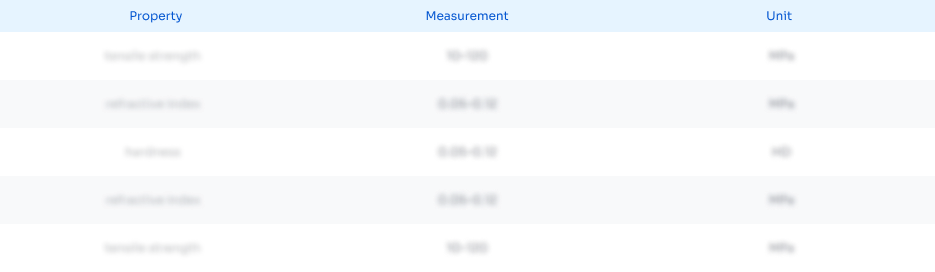
Abstract
Description
Claims
Application Information
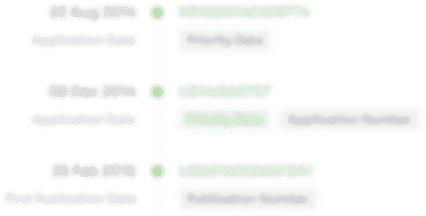
- R&D Engineer
- R&D Manager
- IP Professional
- Industry Leading Data Capabilities
- Powerful AI technology
- Patent DNA Extraction
Browse by: Latest US Patents, China's latest patents, Technical Efficacy Thesaurus, Application Domain, Technology Topic, Popular Technical Reports.
© 2024 PatSnap. All rights reserved.Legal|Privacy policy|Modern Slavery Act Transparency Statement|Sitemap|About US| Contact US: help@patsnap.com