Weak supervision semantic segmentation method based on attention directing inference network
A semantic segmentation and network-guiding technology, applied in neural learning methods, biological neural network models, instruments, etc., can solve problems such as blurred target segmentation
- Summary
- Abstract
- Description
- Claims
- Application Information
AI Technical Summary
Problems solved by technology
Method used
Image
Examples
Embodiment Construction
[0033] It should be noted that, in the case of no conflict, the embodiments in the present application and the features in the embodiments can be combined with each other. The present invention will be further described in detail below in conjunction with the drawings and specific embodiments.
[0034] figure 1 It is a system flowchart of a weakly supervised semantic segmentation method based on guided attention reasoning network of the present invention. Mainly consists of self-guiding of network attention and integrating additional supervision.
[0035] Since the attention map reflects the regions on the input image that support the network's predictions, a guided attention inference network is proposed, whose purpose is to supervise the attention map when training the network for the task of interest.
[0036] GAIN forms constraints directly on the attention map in a regularized guided manner; GAIN has two network streams: classification stream S cl and attention mining S...
PUM
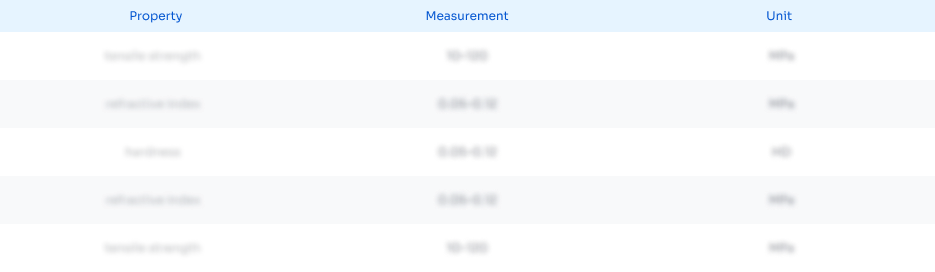
Abstract
Description
Claims
Application Information
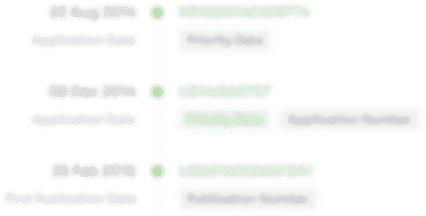
- R&D Engineer
- R&D Manager
- IP Professional
- Industry Leading Data Capabilities
- Powerful AI technology
- Patent DNA Extraction
Browse by: Latest US Patents, China's latest patents, Technical Efficacy Thesaurus, Application Domain, Technology Topic.
© 2024 PatSnap. All rights reserved.Legal|Privacy policy|Modern Slavery Act Transparency Statement|Sitemap