Network traffic classification method based on K_means and KNN fusion algorithm
A fusion algorithm and network traffic technology, applied in the field of network traffic classification, Qos and unlogged traffic identification, network management, can solve the problems of unrecognized unlogged traffic, single form of network traffic classification method, low accuracy rate, etc., to achieve high Classification accuracy and recall, improved classification performance, functional and performance improvements
- Summary
- Abstract
- Description
- Claims
- Application Information
AI Technical Summary
Problems solved by technology
Method used
Image
Examples
Embodiment Construction
[0043] The technical solution of the present invention will be clearly and completely described below in conjunction with the accompanying drawings in the present invention.
[0044] As attached figure 1 As shown, the present invention proposes a network traffic classification method based on K_means and KNN fusion algorithm. The overall framework is divided into two stages: feature selection and (N+1) classification model. In the feature selection stage, the present invention proposes a feature selection method based on iterative K_means to select the optimal feature subset for each application category; in the (N+1) classification model stage, N two classifiers are established, and the decision rules The final classification result is output after integrating the N results.
[0045] Step 1: Collect the flow data set. Download the MAWI network public data set, manually capture the traffic of various application categories locally to generate a local data set. Use Wireshark too...
PUM
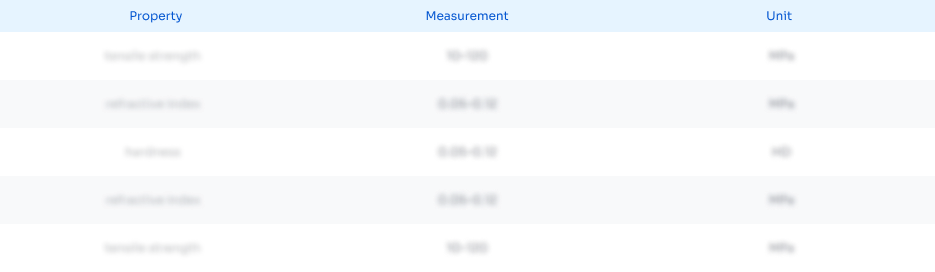
Abstract
Description
Claims
Application Information
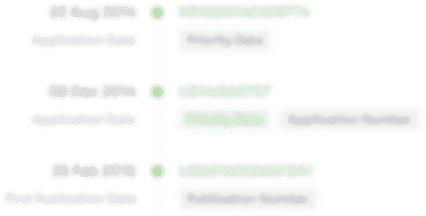
- R&D
- Intellectual Property
- Life Sciences
- Materials
- Tech Scout
- Unparalleled Data Quality
- Higher Quality Content
- 60% Fewer Hallucinations
Browse by: Latest US Patents, China's latest patents, Technical Efficacy Thesaurus, Application Domain, Technology Topic, Popular Technical Reports.
© 2025 PatSnap. All rights reserved.Legal|Privacy policy|Modern Slavery Act Transparency Statement|Sitemap|About US| Contact US: help@patsnap.com