Image color expression mode migration method based on deep convolutional neural networks
A deep convolution and neural network technology, applied in the field of deep learning, can solve problems such as incomplete separation of image content and style, distortion of the resulting image structure, and destruction of natural image structure information.
- Summary
- Abstract
- Description
- Claims
- Application Information
AI Technical Summary
Problems solved by technology
Method used
Image
Examples
Embodiment Construction
[0096] The present invention will be further described in detail below in conjunction with specific embodiments, which are explanations of the present invention rather than limitations.
[0097] The present invention extracts the style features of the image by using a deep convolutional neural network pre-trained in the image style recognition task. The network can extract image color features more efficiently. The VGG-19 (or VGG-16) network introduces too much structural information in the image style feature extraction, so that the generated result image destroys the structural information of the content image, causing distortion problems.
[0098] A deep convolutional neural network pre-trained for image style recognition tasks, which is trained in the task of distinguishing images from natural images and impressionist painting images, can represent image color pattern features more efficiently. The structure of the specific deep convolutional neural network proposed in the p...
PUM
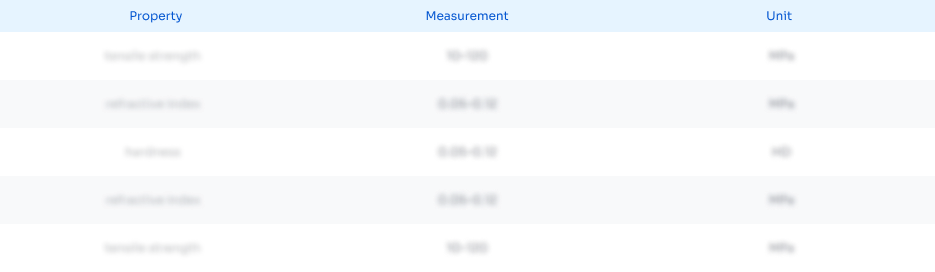
Abstract
Description
Claims
Application Information
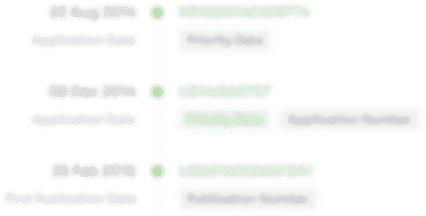
- R&D Engineer
- R&D Manager
- IP Professional
- Industry Leading Data Capabilities
- Powerful AI technology
- Patent DNA Extraction
Browse by: Latest US Patents, China's latest patents, Technical Efficacy Thesaurus, Application Domain, Technology Topic, Popular Technical Reports.
© 2024 PatSnap. All rights reserved.Legal|Privacy policy|Modern Slavery Act Transparency Statement|Sitemap|About US| Contact US: help@patsnap.com