Image feature extraction and training method based on three-dimensional convolutional neural network
An image feature extraction and three-dimensional convolution technology, applied in the field of image recognition and deep learning, can solve the problems of large amount of calculation, low recognition, loss of information, etc., to improve the accuracy, improve the recognition rate, and optimize the training effect.
- Summary
- Abstract
- Description
- Claims
- Application Information
AI Technical Summary
Problems solved by technology
Method used
Image
Examples
Embodiment Construction
[0039] The present invention will be further described in detail below in conjunction with specific embodiments, which are for explanation rather than limitation of the present invention.
[0040] The present invention is an image feature extraction and training method based on a three-dimensional convolutional neural network. The method constructs a three-dimensional convolutional neural network model and a corresponding training method. It is different from the previous two-dimensional convolutional neural network method. The image needs to average or divide the information of a certain dimension in 3D into many channels, so 3D features cannot be extracted effectively. This method directly uses 3D convolution to extract 3D features, and uses proportional equalization when training sample models The standardized small-batch sample input mechanism estimates the gradient, avoiding the disadvantage that some sample categories cannot be effectively identified due to random input samp...
PUM
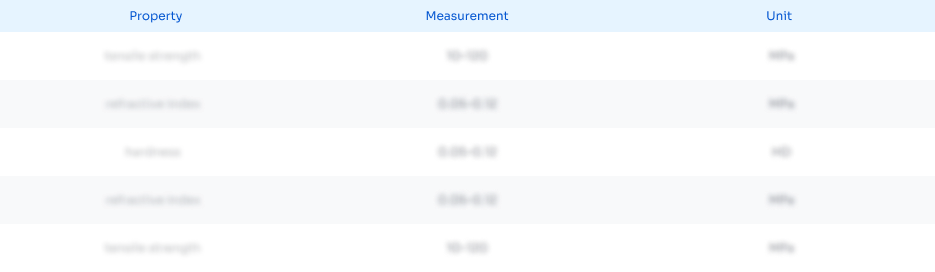
Abstract
Description
Claims
Application Information
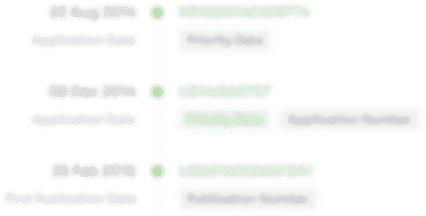
- R&D
- Intellectual Property
- Life Sciences
- Materials
- Tech Scout
- Unparalleled Data Quality
- Higher Quality Content
- 60% Fewer Hallucinations
Browse by: Latest US Patents, China's latest patents, Technical Efficacy Thesaurus, Application Domain, Technology Topic, Popular Technical Reports.
© 2025 PatSnap. All rights reserved.Legal|Privacy policy|Modern Slavery Act Transparency Statement|Sitemap|About US| Contact US: help@patsnap.com