A dynamic process monitoring method based on bp neural network autoregressive model
A technology of BP neural network and autoregressive model, which is applied in biological neural network models, neural learning methods, neural architectures, etc., and can solve problems such as which data cannot be sampled into
- Summary
- Abstract
- Description
- Claims
- Application Information
AI Technical Summary
Problems solved by technology
Method used
Image
Examples
Embodiment Construction
[0049] The method of the present invention will be described in detail below in conjunction with the accompanying drawings and specific examples of implementation.
[0050] Such as figure 1 As shown, the present invention discloses a dynamic process monitoring method based on BP neural network autoregressive model. The specific implementation process of the method of the present invention will be described below in conjunction with an example of a specific industrial process.
[0051] Before introducing specific implementation cases, it is necessary to briefly introduce the basic principles of the BP neural network. Let the input mode of BP neural network be α=[α 1 , α 2 ,...,α dm ], the output of the hidden layer is β=[β 1 , β 2 ,...,β 2dm ], the output of the output layer is γ=[γ 1 , gamma 2 ,…, γ m ], the actual output value of the neural network is y=[y 1 ,y 2 ,...,y m ]. According to the principle of BP neural network, the hidden layer output β can be obtain...
PUM
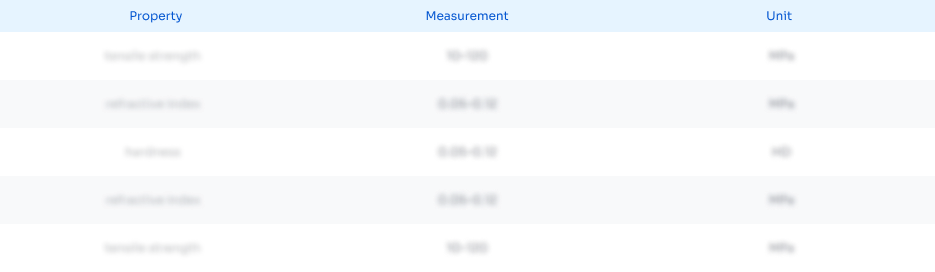
Abstract
Description
Claims
Application Information
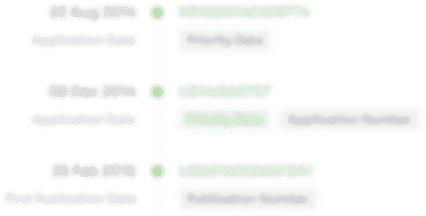
- R&D Engineer
- R&D Manager
- IP Professional
- Industry Leading Data Capabilities
- Powerful AI technology
- Patent DNA Extraction
Browse by: Latest US Patents, China's latest patents, Technical Efficacy Thesaurus, Application Domain, Technology Topic, Popular Technical Reports.
© 2024 PatSnap. All rights reserved.Legal|Privacy policy|Modern Slavery Act Transparency Statement|Sitemap|About US| Contact US: help@patsnap.com