A home design method based on deep neural network
A deep neural network and home design technology, applied in the field of deep learning, can solve problems such as design styles that cannot be learned, and achieve a more intelligent effect
- Summary
- Abstract
- Description
- Claims
- Application Information
AI Technical Summary
Problems solved by technology
Method used
Image
Examples
Embodiment 1
[0041] A home design method based on a deep neural network. Firstly, a sequential structure home prediction model based on dependency features is proposed, and the model is designed and used to extract the structural features between home characters and learn the prediction of the order placement sequence. The specific implementation steps are as follows:
[0042] Step 1. Labeling of household datasets and collation of datasets
[0043]The home design display is collected from professional website home design drawings and home floor plans. The standard used in the pictures is that the space is relatively complete and the home display meets the medium-sized house specifications. The homes involved are marked, and the choice of home labeling tends to be volume. Objects that are relatively large and individually independent, use English words to indicate the category of objects, and household objects are placed around the wall for a week, and the order of these household furnishi...
Embodiment 2
[0060] A home design method based on a deep neural network, which further introduces parameter constraints that limit the relative size of home objects, and builds a two-way layered home prediction model. In the prediction stage, cluster search is used to screen parameters, and the scheme design of the home sequence is completed. The model flow is as follows: figure 2 shown. The specific implementation steps are as follows:
[0061] Step 1: Construction of a hierarchical phrase-level household data set
[0062] Add a number after each home character, that is, the size of the number indicates the size of the home. Here it indicates the length of the wall relative to it. For each type of home, set several different sizes that are commonly used; Next To construct a hierarchical input and output pair, due to the introduction of size parameters, each wall needs to be predicted separately, and a pair of input and output sequence pairs in a non-sequential structure constitutes a c...
PUM
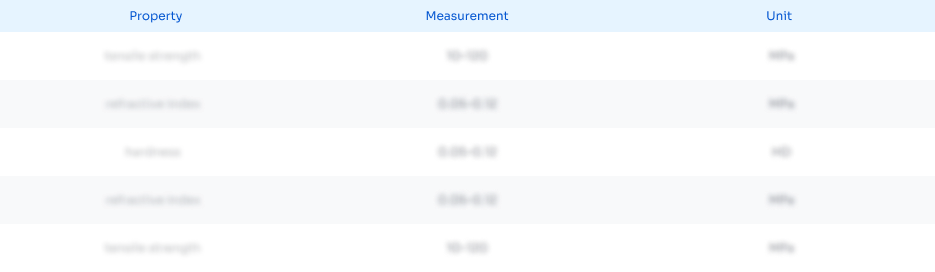
Abstract
Description
Claims
Application Information
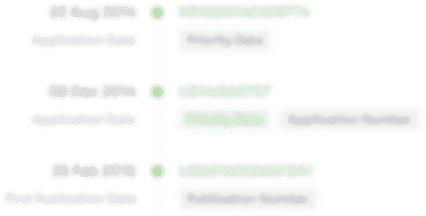
- R&D
- Intellectual Property
- Life Sciences
- Materials
- Tech Scout
- Unparalleled Data Quality
- Higher Quality Content
- 60% Fewer Hallucinations
Browse by: Latest US Patents, China's latest patents, Technical Efficacy Thesaurus, Application Domain, Technology Topic, Popular Technical Reports.
© 2025 PatSnap. All rights reserved.Legal|Privacy policy|Modern Slavery Act Transparency Statement|Sitemap|About US| Contact US: help@patsnap.com