Neural fuzzy Wiener-Hammerstein model identification method based on combined signal source
A neuro-fuzzy and model identification technology, applied in the field of Wiener-Hammerstein identification, can solve problems such as the number of iterations cannot be effectively guaranteed, the algorithm is complicated, etc., to avoid parameter initialization and convergence is difficult to be guaranteed, solve unmeasurable problems, strong The effect of a linear process
- Summary
- Abstract
- Description
- Claims
- Application Information
AI Technical Summary
Problems solved by technology
Method used
Image
Examples
Embodiment Construction
[0026] In order to enable those skilled in the art to better understand the solutions of the present invention, the technical solutions in the embodiments of the present invention will be clearly and completely described below in conjunction with the drawings in the embodiments of the present invention.
[0027] refer to figure 1 , in an embodiment of the present invention, a neuro-fuzzy Wiener-Hammerstein model identification method based on a combined signal source is provided, and the identification method includes the steps of:
[0028] S1: Construct a single-input and single-output Wiener-Hammerstein model formed by the series connection of input dynamic linear link, output dynamic linear link and static nonlinear link; S2: Gaussian signal and binary signal are combined to form a multi-signal source as the Wiener-Hammerstein model. The input of the Hammerstein model; and S3: separate and identify the input dynamic linear link, the output dynamic linear link and the static...
PUM
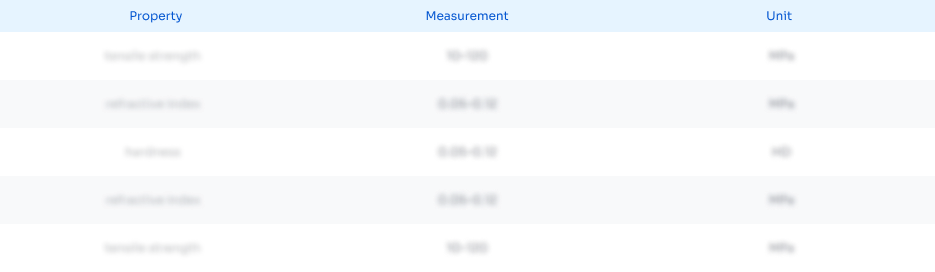
Abstract
Description
Claims
Application Information
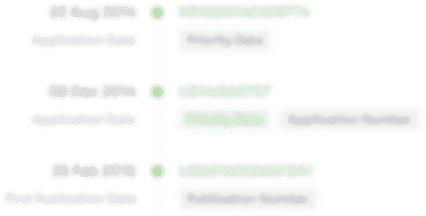
- R&D Engineer
- R&D Manager
- IP Professional
- Industry Leading Data Capabilities
- Powerful AI technology
- Patent DNA Extraction
Browse by: Latest US Patents, China's latest patents, Technical Efficacy Thesaurus, Application Domain, Technology Topic, Popular Technical Reports.
© 2024 PatSnap. All rights reserved.Legal|Privacy policy|Modern Slavery Act Transparency Statement|Sitemap|About US| Contact US: help@patsnap.com