First-person-view gesture recognition method based on dynamic image and video subsequence
A dynamic image and first-person technology, applied in the field of first-person perspective gesture recognition, can solve the problems of complex extraction process and low precision, and achieve the effect of improving recognition accuracy, improving precision, and improving processing speed
- Summary
- Abstract
- Description
- Claims
- Application Information
AI Technical Summary
Problems solved by technology
Method used
Image
Examples
Embodiment 1
[0085] Such as Figure 1-5 As shown, the implementation is as follows:
[0086] Step 1: Collect color video and depth video, and preprocess the video to obtain video data;
[0087] Step 2: Construct dynamic images and extract video representative sequences based on video data;
[0088] Step 3: Input dynamic images and video representative sequences into the fine-tuned neural network model to obtain features, and input the features into the SVM classifier to obtain category probabilities;
[0089] Step 4: Fuse category probabilities to complete gesture recognition.
[0090] Fine-tuning of neural network modules for dynamic image features:
[0091] After the network is built, it is trained. Since the sample data for gesture recognition is insufficient, the fine-tuning model is used. The fine-tuning model selects a model that is pre-trained on a large-scale data set such as ImageNet, and then fine-tunes the pre-trained model on the new data set. ;The pre-training model contai...
Embodiment 2
[0096] Such as Figure 1-5 As shown, the implementation is as follows:
[0097] The preprocessing in step 1 includes conventional preprocessing of the depth video and preprocessing of the color video after gesture segmentation; the preprocessing includes grayscale of the video frame to obtain a grayscale image, dilation and hole filling of the grayscale image, and Convert a grayscale image to a binary image.
[0098] Step 2 includes the following steps:
[0099] Step 2.1: Weight and sum each frame of video data to obtain a dynamic image;
[0100] Step 2.2: The video data adopts the extraction method based on the frame difference to obtain the video representative sequence.
[0101] The extraction method based on frame difference adopted in step 2.2, the specific steps are as follows:
[0102] Step a: Calculate the frame difference and obtain the maximum value of the frame difference;
[0103]Step b: Determine whether the two frames corresponding to the maximum frame diffe...
PUM
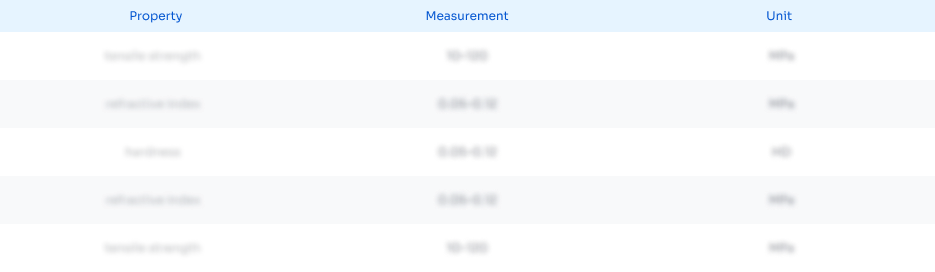
Abstract
Description
Claims
Application Information
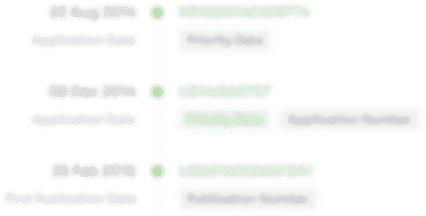
- R&D
- Intellectual Property
- Life Sciences
- Materials
- Tech Scout
- Unparalleled Data Quality
- Higher Quality Content
- 60% Fewer Hallucinations
Browse by: Latest US Patents, China's latest patents, Technical Efficacy Thesaurus, Application Domain, Technology Topic, Popular Technical Reports.
© 2025 PatSnap. All rights reserved.Legal|Privacy policy|Modern Slavery Act Transparency Statement|Sitemap|About US| Contact US: help@patsnap.com