Image classification method for chronic venous disease based on multi-scale semantic features
A semantic feature and classification method technology, applied in the field of medical image classification, can solve the problems of no overall consideration, poor performance of single local feature discrimination, ignoring local label information, etc., to achieve the effect of narrowing the semantic gap and high accuracy
- Summary
- Abstract
- Description
- Claims
- Application Information
AI Technical Summary
Problems solved by technology
Method used
Image
Examples
Embodiment Construction
[0024] In order to make the object, technical solution and advantages of the present invention clearer, the present invention will be further described in detail below in conjunction with the accompanying drawings and embodiments. It should be understood that the specific embodiments described here are only used to explain the present invention, not to limit the present invention. In addition, the technical features involved in the various embodiments of the present invention described below can be combined with each other as long as they do not constitute a conflict with each other.
[0025] Such as figure 1 As shown, an image classification method for chronic venous diseases based on multi-scale semantic features, including:
[0026] (1) Carry out multi-scale division of the image of chronic venous disease to be tested. On each scale, use the concept classifier corresponding to each scale to classify the image blocks in the image of chronic venous disease to be tested, and ...
PUM
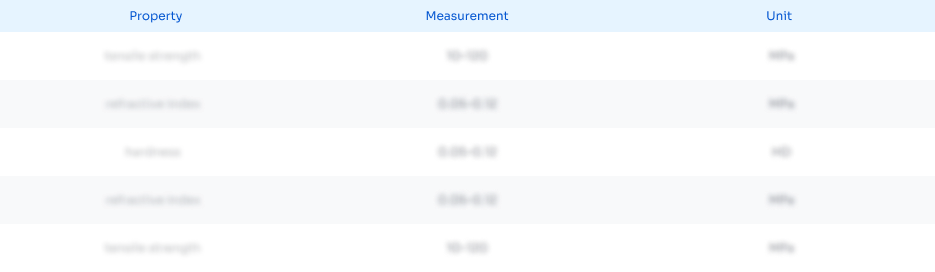
Abstract
Description
Claims
Application Information
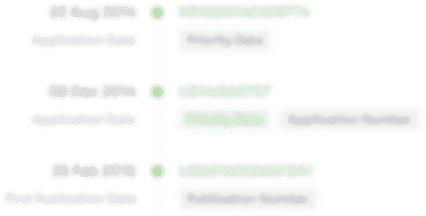
- R&D Engineer
- R&D Manager
- IP Professional
- Industry Leading Data Capabilities
- Powerful AI technology
- Patent DNA Extraction
Browse by: Latest US Patents, China's latest patents, Technical Efficacy Thesaurus, Application Domain, Technology Topic, Popular Technical Reports.
© 2024 PatSnap. All rights reserved.Legal|Privacy policy|Modern Slavery Act Transparency Statement|Sitemap|About US| Contact US: help@patsnap.com