Universal cross-modal retrieval model based on deep hash
A cross-modal and model technology, applied in network data retrieval, other database retrieval, biological neural network models, etc., can solve the problems of model retrieval delay and inefficiency, large storage space, and ignoring retrieval efficiency.
- Summary
- Abstract
- Description
- Claims
- Application Information
AI Technical Summary
Problems solved by technology
Method used
Image
Examples
Embodiment 1
[0030] Such as figure 1 As shown, a general cross-modal retrieval model based on deep hashing, the model includes image model 1, text model 2, binary code conversion model 3 and Hamming space 4, in which:
[0031] Image model 1, extracting image features, abstracting original image features and semantics;
[0032] Text model 2, which converts text data into vector form, and extracts the features and semantics of the text;
[0033] Binary code conversion model 3, which converts the features and semantics extracted by the image and text models into binary codes, and then maps the data points in the original feature space of different modalities to the common Hamming space;
[0034] Hamming space 4, the common subspace of the image and text modal feature space, in which the Hamming distance between the query data and the original data binary hash code is calculated for similarity ranking.
[0035] The image model 1 mainly recommends the use of CNN models such as ResNet, DenseNe...
PUM
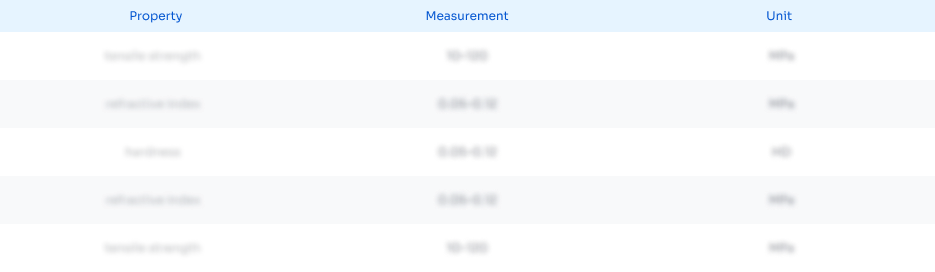
Abstract
Description
Claims
Application Information
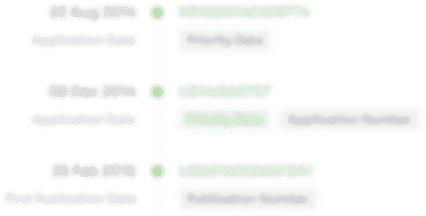
- R&D Engineer
- R&D Manager
- IP Professional
- Industry Leading Data Capabilities
- Powerful AI technology
- Patent DNA Extraction
Browse by: Latest US Patents, China's latest patents, Technical Efficacy Thesaurus, Application Domain, Technology Topic, Popular Technical Reports.
© 2024 PatSnap. All rights reserved.Legal|Privacy policy|Modern Slavery Act Transparency Statement|Sitemap|About US| Contact US: help@patsnap.com