A multi-graph regularized deep matrix factorization method for multi-view clustering
A matrix decomposition, multi-view technology, applied in the field of image processing, can solve problems such as insufficient accuracy, inability to preserve the manifold structure of each view, and no consideration, to achieve the effect of improving accuracy, eliminating adverse effects, and effectively and rationally optimizing
- Summary
- Abstract
- Description
- Claims
- Application Information
AI Technical Summary
Problems solved by technology
Method used
Image
Examples
Embodiment Construction
[0038] In order to more clearly describe the embodiments of the present invention or the technical solutions in the prior art, the specific embodiments of the present invention will be described below with reference to the accompanying drawings. Obviously, the accompanying drawings in the following description are only some embodiments of the present invention. For those of ordinary skill in the art, other drawings can also be obtained from these drawings without creative efforts, and obtain other implementations.
[0039] For the semi-nonnegative matrix (Deep Semi-NMF) decomposition, the objective function is where X∈IR m×n Represents the input data of n samples, each sample is an m-dimensional feature, A ∈ IR m×r , W ∈ IR r×n , W≥0, where W represents the “soft” clustering assignment matrix. While in reality, natural data may contain different pattern connections (or factors), for example, expressing the effect of lighting on a face dataset, a single non-negative matrix...
PUM
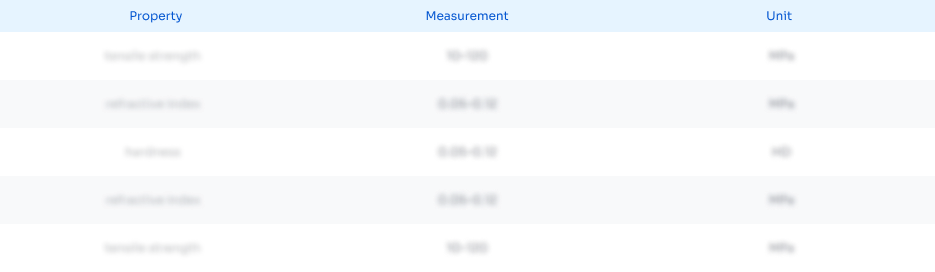
Abstract
Description
Claims
Application Information
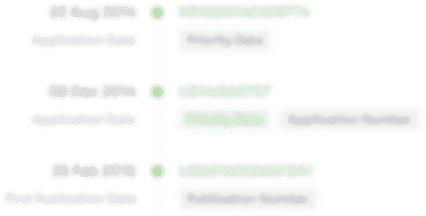
- R&D Engineer
- R&D Manager
- IP Professional
- Industry Leading Data Capabilities
- Powerful AI technology
- Patent DNA Extraction
Browse by: Latest US Patents, China's latest patents, Technical Efficacy Thesaurus, Application Domain, Technology Topic.
© 2024 PatSnap. All rights reserved.Legal|Privacy policy|Modern Slavery Act Transparency Statement|Sitemap